The data scientists were baffled. They had been training the algorithm for weeks, watching it reach new heights of accuracy when identifying dog breeds. 85 percent, 95 percent, and eventually it peaked at 98 percent accuracy. However, every now and then, it seemed to throw the same error, identifying a multitude of very distinct breeds as the same thing: a husky. Repeatedly, the model would return husky when the picture was clearly of a poodle, a dalmatian, even a bulldog. Frustrated, they reverse engineered explainability into the model.
The result was shocking.
The algorithm was completely ignoring the features of the animals in the pictures. Instead, to the data scientists’ collective surprise, it was using other attributes of the image that happened to be common to every husky picture they had used to train it. It was identifying the picture because of the snow and the trees in the background.
Imagine if the machine learning (ML) algorithm that processed your mortgage application was using equally irrelevant attributes to make a critical decision for your future, such as the gender or ethnic origin you declared on the application form.
There is no question that machine learning-based models are critical enablers for the complex processes inherent in the modern world, however, organizations are becoming increasingly aware of the impacts from getting their implementation even slightly wrong. And high-profile examples, including the well-publicized reputational impact suffered by Apple as a result of the gender bias in the credit limits offered during its credit card launch and the $100 billion market value impact suffered by Google after the launch of its GPT offering, Bard, have ensured that the public are too.
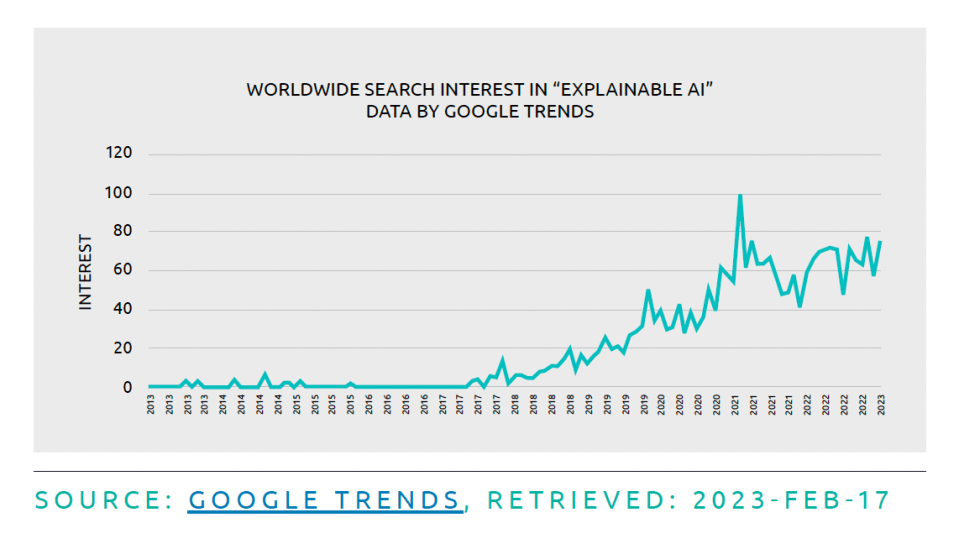
Can you really see me?
Customer intimacy is a gauge of your alignment with your customers’ needs and values. It’s more than just talking to your customers; it’s about understanding them and understanding their perception of your organization. Being customer-centric relies on cultivating customer intimacy, and that relationship is built on trust. Why am I being offered that particular promotion? Why have I been quoted a particular price for my online purchase or had a particular video suggested to me? In the early days of AI mass-adoption, from the mid-2010s (sometimes termed the “AI summer”), consumers and customers were largely willing to accept the “magic” of artificial intelligence on face value. However, as machine-learning algorithms have become progressively integrated into our interactions with technical systems, service providers, and even other people, terms like “interpretable ML,” “explainable AI,” and “glass-box model” have become increasingly pertinent to this key group. This demand for transparency from customers, consumers, and citizens alike is only going to be further amplified as their digital dexterity and AI literacy increases, supported by the increasing focus on customer experience and the simplification introduced though automation and AI.
“Being customer-centric relies on cultivating customer intimacy, and that relationship is built on trust.”
20:20 vision
Legislation is catching up; just consider the explainability clauses proposed in the EU and UK’s forthcoming AI strategies, or even just the tenets of GDPR, but irrespective of how much an organization fears falling afoul of some current or future law, good corporate governance is a critical discipline; it is an enabler and liberator for an organization when it is done properly and, conversely, an inhibitor for corporate growth when it isn’t. Having clear control of the organization’s processes is at the core of getting governance right, so understanding the AI that is powering them should not be considered optional.
Investigating and validating modern end-to-end ML is akin to opening the hood of a modern car. With some basic guidance, we can identify the major components but that’s about it for most people. Knowing how all those components propel your vehicle is as inscrutable as understanding just why a hotel room in Croydon suddenly costs so much more on a particular day on that travel booking site. And while, in the case of a car, there are well-understood laws, rigorous tests, and certifications that ensure the vehicle’s road worthiness, those tests and certifications are not yet formally in place for AI systems. Yet. For the most part, ML models remain unregulated, custom-designed information processing systems that we have little understanding of and are too often referred to as “black boxes.”
Opening the black box
For those reasons, a black-box AI system is no longer adequate for a competitive organization, especially when there are increasingly compelling requirements for proactive responsibility, compliance, and sustainability targets. Major technology industry players have themselves introduced open-source toolkits for assessing biases and explaining model behavior, with Microsoft’s Fairlearn and IBM’s AI Fairness 360 being the most prominent examples.
Almost every ML project we undertake involves work on explainability, because it allows us to rationalize model behavior when presenting results to our clients. And thanks to the advances in explainable AI, we have seen a resurgence of interest in previously niche research areas such as causal ML, fair ML, ethical AI, and sustainable AI. Taking the latter as an example, using explainable AI techniques to better understand what a model is actually doing will lift the lid on whether the additional power consumption required, that “carbon investment,” is really worthwhile if it only improves the accuracy of the model by a few percentage points.
It is true that AI is currently allowing us to explore information use that we never previously thought possible (one of the authors is really looking forward to AI-generated unit tests). Nevertheless, that exploration needs to entail the introspection and explainability of the AI systems themselves, so that we can be certain that everyone benefits from them. In the very near future, as AI becomes increasingly pervasive across all facets of society, people are going to want to know exactly what you think you know about them, and exactly how you are using that information to make decisions on their behalf. Expect an increasing number of questions like this and be prepared to be able to answer them with good explainable AI practices.
INNOVATION TAKEAWAYS
EXPLAINABLE AI IS IN DEMAND
The public are already demanding that the decisions organizations make with AI are explainable and appropriately transparent.
CONSUMER SENTIMENT IS CHANGING
As digital dexterity and AI literacy increases, people are no longer willing to accept the “magic” of algorithms on face value.
THE TIME TO ACT IS NOW
Implementing explainable practices in AI and ML will provide much-needed trust and customer intimacy.
Interesting read?
Capgemini’s Innovation publication, Data-powered Innovation Review | Wave 6 features 19 such fascinating articles, crafted by leading experts from Capgemini, and key technology partners like Google, Starburst, Microsoft, Snowflake and Databricks. Learn about generative AI, collaborative data ecosystems, and an exploration of how data an AI can enable the biodiversity of urban forests. Find all previous waves here.
“Capgemini partners with companies to transform and manage their business by unlocking the value of technology.
As a leading strategic partner to companies around the world, we have leveraged technology to enable business transformation for more than 50 years. We address the entire breadth of business needs, from strategy and design to managing operations. To do this, we draw on deep industry expertise and a command of the fast-evolving fields of cloud, data artificial intelligence, connectivity, software, digital engineering, and platforms.”
Please visit the firm link to site