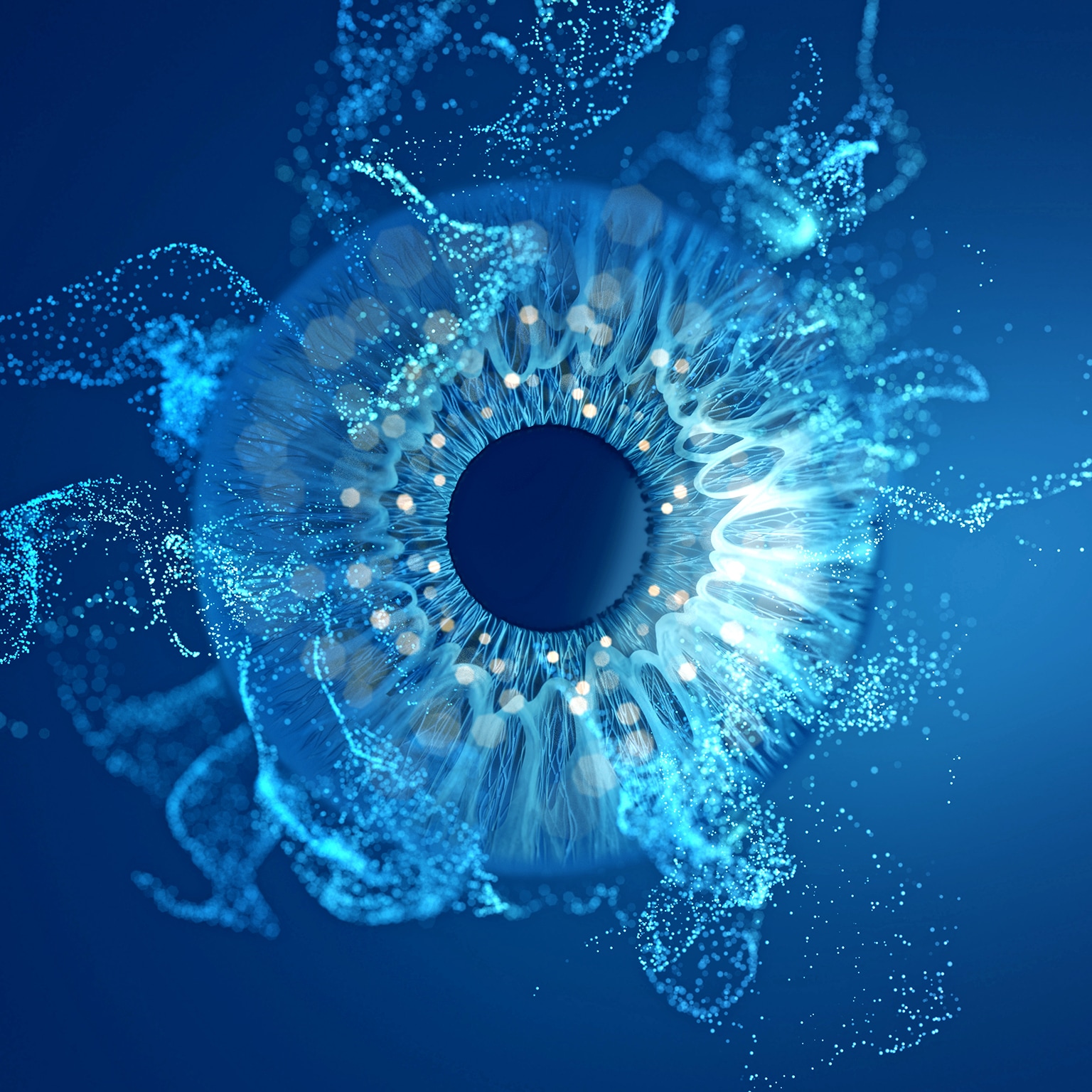
Generative AI (gen AI) is an advancement that has seized the attention of governments, the public, and business leaders. But it presents unique challenges to the professionals tasked with managing gen AI’s existing and potential threats. In this episode of the Inside the Strategy Room podcast, Ida Kristensen, coleader of McKinsey’s Risk & Resilience Practice, and Oliver Bevan, a leader of enterprise risk management, speak with Sean Brown, global director of marketing and communications for the Strategy & Corporate Finance Practice, about the ways business leaders should approach the rapidly evolving impact of the technology.
This is an edited transcript of their conversation. For more discussions on the strategy issues that matter, follow the series on your preferred podcast platform.
Sean Brown: Given how recent this technology is, it’s amazing how much of an evolution we’ve seen and how much development and deployment. But as with all new technologies, there are risks—and maybe more so with this one than with some others. Ida, are you finding your clients widely embracing gen AI?
Ida Kristensen: We see the early adopters that are incredibly excited. We also see many that are quite skeptical and say, “Ah, you know, maybe we’ll wait this one out.” At McKinsey, we are very much in the camp of saying, “Gen AI is here to stay. It offers incredible strategic opportunities across industries and across almost every aspect of what businesses do.”
There is a path to extracting amazing benefits. We also believe that the idea of waiting this one out is not feasible. This is becoming a strategic imperative. Given we are talking about this from a risk angle, another way to say the same thing is that there is a substantial strategic risk associated with not diving in.
That said, there are some real risks associated with deploying gen AI. For an organization to be successful, it requires a defensive, as well as offensive, strategy.
Sean Brown: So, Oliver, given all these risks, what are the regulatory dynamics that companies need to be mindful of?
Oliver Bevan: It’s important to understand how different jurisdictions are taking different approaches to thinking about the risks of gen AI and how they want to govern and approach the overall management of them.
This is importantly different from what we saw in the early days of data privacy. With data privacy, there was a sense that GDPR [General Data Protection Regulation] really led the way. A lot of our clients had adapted their initial data privacy frameworks to GDPR and then thought about adapting them globally using GDPR as a foundation.
We saw a lot of legislation that followed in the steps of GDPR. Some of what was happening in California drew directly on GDPR as well. Our sense is that’s going to be much trickier to do with gen AI.
It’s also clear to a lot of the public sector how much value is at stake and how much AI can directly affect citizens. Obviously, the risks are across data privacy, cybersecurity, and consent around deepfakes, which can have a significant impact on elections and other public-facing events.
That’s why many public actors have taken much more of a front foot approach to thinking about gen AI and, in turn, the responses that organizations need to have. It’s especially true if you’re operating across multiple jurisdictions and thinking about regulatory change. Adapting and embedding these responses into your approach is going to be incredibly important.
Sean Brown: How do the risks and the regulatory landscape differ between gen AI and some of the other advances in AI, including machine learning?
Oliver Bevan: My perspective is that analytical AI, the AI development behind machine learning prior to the adoption of gen AI, is basically anchored in two primary places.
One is data privacy, so that’s considerations around, “How are these models using data? How are you combining data to do synthetic analysis or increase the potential for outputs?”
The second is really on questions around fairness in the model structure. We’re all aware unfortunately that these models tend to have very different outcomes, depending on whether you are looking at different skin tones or different genders. Analytical AI triggered many concerns, especially in the US, around fair housing legislation and optimizing credit decisions in financial services.
Now, there is an increasing awareness of the challenges that come from explainability, for example.
Obviously, there is potential for deepfakes and malicious use of gen AI as the technology can create compelling and realistic facsimiles of either individual identities or corporate identities, which creates massive reputational risks and challenges for governments as well.
Ida Kristensen: It’s a fast evolution, but it is still an evolution of the risks that have been around for a long time, with a couple of twists.
There is the fairness aspect: in most industries, part of the solution has been creating real transparency about how a model works. If you have a more traditional analytical model, whether you regulate it or not, you can explain exactly what happened. That’s part of the reason you should get comfortable with the model.
It is a very different game with gen AI, because the explainability of these models is just, let’s be honest, not quite there. While the risks might be the same, it’ll be very interesting to see how different companies and regulators are going to get comfortable with something that can’t be opened and dissected—where we’ve got to rely on other indicators to get comfortable with fairness.
Sean Brown: Are you seeing any common areas that governments and regulators are focusing on in terms of risk? Or is it primarily explainability?
Oliver Bevan: The most important one is a deeper understanding of how these models work and how these actors are going to have confidence that the models are producing outcomes that can be explained in some fashion.
Explainability is a foundational challenge with gen AI models, as well as sourcing. There is a lot of discussion now about watermarks on gen AI. Can you tell when something’s been generated by AI versus generated by our traditional creative process?
That flows into intellectual property challenges. I suspect, given the raft of elections that are coming up, there’s going to be a lot of focus on those dynamics from the public sector. It’s also incredibly important to think about public trust in these systems and public willingness to use and engage them.
Ida Kristensen: No one will be shocked by our saying that it’s still early days for regulation. We expect much more to come, including sector-specific regulation for regulated industries. There’s no doubt that financial services will face more specific regulation on top of this. So thinking about themes is the right way to do it. Trying to optimize what’s already out there will be a very short-lived strategy.
Sean Brown: For those companies that have been at this for a while, what principles are they following to make sure they use gen AI safely?
Ida Kristensen: For one, don’t let the machines run wild on their own. There’s always a human aspect involved in that. You use the outcome of the models as an input to human decisioning, not as the final decision.
The good news is, gen AI makes it a lot easier to do a lot of rapid tests for fairness. Gen AI can be a real source for good—also in terms of putting in place strong risk management capabilities. Maybe the biggest step change from the responsible AI programs that most organizations have already been working on is the transparency and explainability we talked about already, and then monitoring and evaluation.
It’s a different beast to keep an eye on gen AI’s evolution over time. Therefore, we see clients we work with investing in monitoring gen AI and ask, “Okay, what are the extra bells and whistles and checks we can put in place to get comfortable with what comes out?”
Sean Brown: Any other risks that are out there? And how should companies think about the full gamut of risks that can emerge with gen AI?
Ida Kristensen: Data privacy aspects and quality. One of the things we did at McKinsey, for instance, was we created a network [database] of all our proprietary knowledge [and more] and used that as the training data for some of our applications. That means we control the quality of the data that comes in, and that gives us a great deal of comfort.
Malicious use has been more headline-grabbing because of deepfakes and scams. Consider a malicious actor, for instance, who may impersonate you and write an email asking your uncle to wire them $1,000 tomorrow. They can write those emails; they can translate them into every language. We’ve all grown aware of our personal risk and become used to spam emails. There used to be telltale signs, right? Bad grammar, bad language, and things that just didn’t quite make sense. But now it is so much easier to create high-quality spam emails using gen AI.
Finally, there is strategic risk and third-party risk. Strategic risk is about where you play and how you competitively place yourself, but it also has all the aspects of the broader societal impact. We all know gen AI uses quite a lot of computational power. How does that mesh with your ESG [environmental, social, and governance] commitments? There are broader employment effects as well.
What does it do to the workforce? How do you think about your commitment to your employees and the shift that gen AI will bring? As we mentioned, this is not a technology that’s going to take away net jobs, but it’s a technology that’s going to change jobs very dramatically.
Those are real questions to grapple with.
Sean Brown: Now, if I understand correctly, what you put into your queries can actually feed the model. Does that mean you need to monitor what your employees are asking gen AI?
Ida Kristensen: Yes, it’s potentially scary, right? Any prompt that is answered becomes part of that body of data as well. So most companies should be worried about what their employees are doing.
You’ve got to educate your employees. You can’t just rely on rules. You’ve got to make sure your employees understand the consequences of any prompt they put into a system.
Sean Brown: I’d love to talk a bit more about what the risk management approach model should look like to cover all these novel risks. How are you advising clients on this?
Oliver Bevan: We basically have four categories. One is principles and guardrails. The second is frameworks. The third is deployment and governance. And the fourth is risk mitigation and monitoring.
On principles and guardrails, it’s incredibly important to have an honest conversation as an executive team about how and where you want to use gen AI, while you’re thinking about segmenting your use cases.
Some examples that come up typically are considerations of the degree to which you want to use gen AI to personalize your marketing—for example, the extent to which you want gen AI to be used in performance evaluations or in directly engaging with your employees.
On frameworks, Ida’s already talked about the taxonomy, so I won’t go into that, except to note that there are different flavors of the risks from gen AI and different ways of thinking about them. Having something that works for your organization is incredibly valuable, because it will help you communicate to your employees how they should be thinking about this.
On risk identification, you must have a handle on what types of risks you’re going to face. What a lot of our clients are doing is starting with lower-risk, easier-implementation use cases. That allows them the time to experiment with the governance standards they put up. It allows them time to run the use cases through something like a risk assessment to understand the typical types of risks that they’re exposed to from the use cases.
Sean Brown: I’d love to understand a bit better how companies should approach mitigation of external risks. Let’s take security threats: what kind of measures should they put in place?
Ida Kristensen: It is a mix of tried and tested risk management techniques building on what already exists, potentially turbocharging a few of them and then adding a few more tools to the tricks.
Employees truly need to understand risk 101 of gen AI to be alert and to identify and spot when things are happening. Get people under the tent.
Security is an area where you must fight gen AI with gen AI. Most organizations now really work through it and say, “How do we use gen AI tools to turbocharge our cyber defense, turbocharge the way we do pin testing, the way we think about the different layers of our security, and make sure that our time to detection is way faster?” The time to detection will need to be faster, and the time from detection to shutting things down will need to be faster. That will be super critical.
Sean Brown: Ida, you mentioned you can use AI to fight AI. How could you use gen AI to find deepfakes, for example? I can envision an email that looks and reads like it’s coming from the CEO, directing you to take certain steps. This starts to seem like something you might see in a movie.
Ida Kristensen: Some of it will be process changes. As I mentioned, you might say, “Hey, we were used to, if an ask comes through an email, you just go do it.” No longer. If there’s a voicemail left for you, company policy is you will never act on that voicemail unless you call someone back and you get verification.
There are controls and oversight you could put in place, but, and this is a big theme of what we’re talking about today, it’ll never be enough. Let’s be honest. Controls always have to go hand in hand with this awareness of our employees. You’ve got to make sure that you have people who say, “That’s a little weird. That guy never leaves me a voicemail.”
Risk management is everyone’s job. It needs to get embedded in the fabric and the culture of how we work together.
Sean Brown: What are the typical practices you see clients adopt as they scale internal use of AI?
Oliver Bevan: Overreliance on a small group of experts. Obviously, at the start when you’re building use cases, you will have potentially limited capabilities internally. You’ll also have a group of acolytes or very excited people who want to spend a lot of their time working on gen AI. That small group of experts is going to rapidly get overwhelmed as you scale gen AI. It’s going to create a lot of friction, a lot of frustration, and it’s going to slow everything down.
Relying solely on vendors is also not something we’d recommend, frankly even at the early stages. There’s wide variation in terms of what the large language model providers and the other third-party ecosystems are doing. You need to take responsibility for diligence on gen AI, and you need to think about what you can do internally beyond just what they offer as out-of-the-box security solutions. Similarly, just having technical mitigation strategies is typically not going to be sufficient.
We’re still learning a lot about how the different controls and mitigations perform. And so you need some overlay of human factors, including things like having a human in the loop. Integrate risk and development groups as soon as you can. Given the evolution and dynamics of gen AI, be aware that these will evolve and change over time and that you need to have ways to track that evolution for successful and sustainable scaling.
Sean Brown: Any final thoughts before we close?
Ida Kristensen: Yes. If in our discussion of risk, it came across as doomsday, we apologize. In my mind, it’s the classic idea of building better brakes to go faster. That’s what it is. In addition, let’s call me a seasoned risk practitioner.
I’m incredibly excited as well about this idea of shifting left. It is something we have talked about for many years—and it’s been hard to implement. We now have enough value at stake that we will begin to see a more seamless collaboration, in an agile way, between risk and the development of gen AI. So, yes, we’ll unleash all this potential from the technology but hopefully also get it right in terms of working together and embedding risk much earlier, which would make an old practitioner like me very happy.
“Our firm is designed to operate as one—a single global partnership united by a strong set of values. We are equally committed to both sides of our mission: attracting and developing a talented and diverse group of colleagues and helping our clients create meaningful and lasting change.
From the C-suite to the front line, we partner with clients to help them innovate more sustainably, achieve lasting gains in performance, and build workforces that will thrive for this generation and the next.”
Please visit the firm link to site