Generative AI’s (GenAI) power to transform every aspect of our lives is now common knowledge
But business leaders are still unsure exactly how to make this revolutionary technology an integral part of their activities. The truth is that the integration of Generative AI for operations requires a very different approach than the integration of traditional AI. This is evident in the sphere of R&D and operations.
In recent years, AI has demonstrated concrete impact across the entire operations value chain. However, the most common and widespread use cases consistently focus on optimizing core processes. One notable development is the way industry leaders use AI to optimize the “physical” manufacturing and delivery of goods. Significant changes include time series analysis to improve process yield or scrap rate, operational research optimizing goods inventory norms, flows of goods or transportation, and computer vision to detect non-conformities.
With its unmatched ability to navigate, digest, and interact with unstructured information and documentation, GenAI reoriented the focus from the “physical” to the “information” world. This means a shift from sensors and connected assets data to documents, which in turn leads to a retreat from manufacturing and supply chain core processes to R&D and industry enabling functions (e.g., sourcing, maintenance, quality and regulatory, etc.).
With this new paradigm in mind, leaders in operations see a new world of opportunities opening up before them, all powered by GenAI .
Key capabilities for R&D and operations
Generative AI for research and operations is a gamechanger for all industries worldwide, optimizing organizations by automating data analysis and customer service tasks. Right now, many organizations are rightly experimenting, developing best practices, and identifying scalable solutions. We at Capgemini Invent were some of the first movers in this space, using our expertise to envision applications.
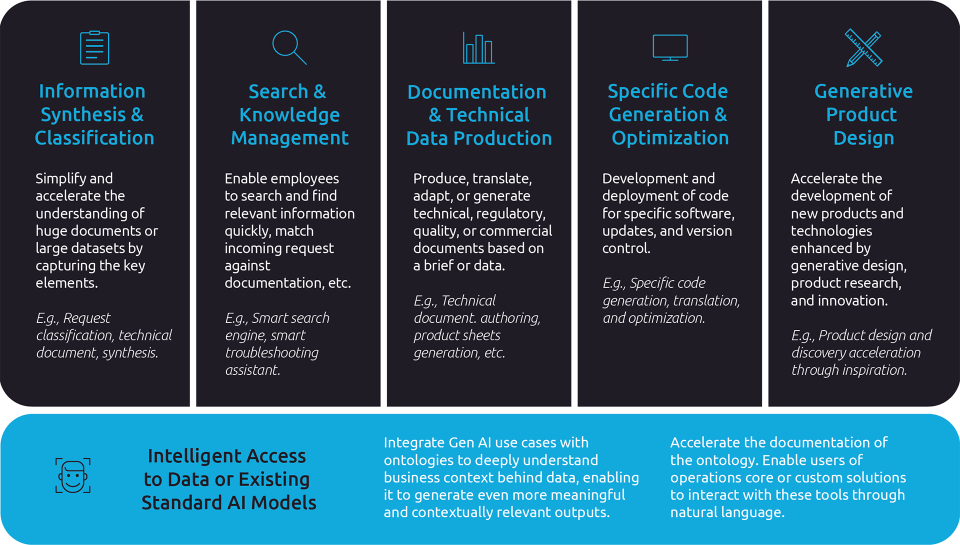
Even though we are only at the dawn of this transformation, several concrete use cases are already shining bright with more emerging every week. All that remains is to explore the untold opportunities.
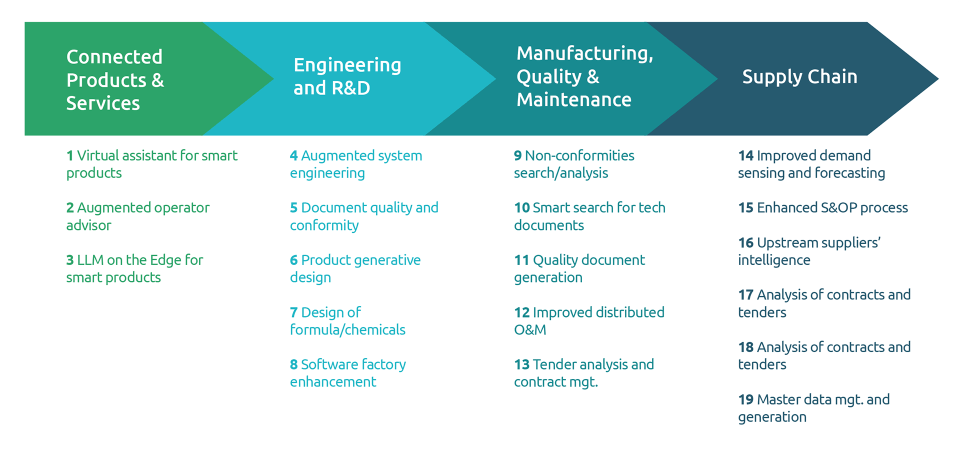
Smart products
The ability of GenAI to emulate a human conversation makes it a prime candidate for enriching user experience with “companion apps”. These apps leverage data collected by connected devices and expose it through a virtual assistant, enabling interactions in natural language and access to insights generated in real time. In the near future, we foresee the emergence of autonomous, edged Large Language Models (LLMs) embedded directly within products, enabling a new range of usage.
Engineering and R&D
As it is probably the most document-intensive area of the industry, GenAI offers a multitude of opportunities for engineering and R&D. The ability of GenAI to digest and synthetize complex information, combined with Retrieval-Augmented Generation (RAG) , enables engineers to easily search and query knowledge base or technical documentation using natural language. LLMs have multiple applications all along the technical documentation lifecycle, accelerating creation of a draft, proofreading or consistency checks against existing standards.
From the innovative use of AI algorithms for Automated Molecule Design in Drug Discovery to the Fragrance Formulation Generator streamlining perfume creation, the applications are far-reaching and go way beyond LLMs. It’s all down to GenAI’s ability to craft detailed 3D simulation scenarios.
Manufacturing quality and maintenance
Leveraging its abilities to extract, synthesize, and classify information, GenAI can optimize and turbocharge several manufacturing, quality, and maintenance processes. For instance, it can accelerate classification, summarization, search and analysis of quality incidents. It can also automate generation of quality documentation in domains with heavy compliance requirements (e.g., life sciences) and automate the documentation of non-conformities.
GenAI-powered software enables human operators in maintenance or engineering to rapidly navigate through documentation of assets in a targeted way. Furthermore, Generative AI in manufacturing and operations will be instrumental in the consolidation of information from other external sources, such as weather forecasts, market insights, and assessment of geopolitical risks. This capacity to integrate data from multiple sources is why GenAI maximizes maintenance planning, accelerates operations, and improves efficiency.
This is all the truer in the case of distributed operations, where data coming from different systems is often heterogeneous and sometimes inconsistent. GenAI’s ability to automatically harmonize and retreat data from distributed environments to ensure it meets quality standards will be key to enable seamless utilization of these data flows and to de-risk associated field operations.
Supply chain and purchasing
GenAI can also significantly increase productivity of interactions across a network of partners, assets, and inventory. More specifically, GenAI can boost the resiliency and efficiency of supply chains in the following ways:
- Enriched demand planning: Complementary to “standards” ML-based forecasting, by analyzing various exogeneous data sources (customer reviews, social-media trends, articles, etc.) to better understand demand drivers.
- Efficient sourcing: For instance, through improved upstream suppliers’ intelligence, by crossing external information regarding the upstream supply chain with the analysis of internal suppliers’ documentation and deriving insights from these data. GenAI can also improve procurement process efficiency by automating transactional tasks involving interactions with suppliers or processing of external documentation (contract analysis, CSR compliance check, automated review of tenders, etc.).
- Improved Customer interactions: Automating and augmenting customer service and back-office operations, by accelerating search, summarization, classification and processing of trade, logistics and customer claims.
Three detailed use cases
The following three case studies provide an idea of how AI will change R&D and operations in the near future.
1. Search, synthesis, and reconciliation of engineering documentation
In the engineering industry, accelerating development processes while securing the quality on most complex products or major industrial projects are key challenges.
The main difficulties usually encountered are:
i) Limited access to engineering knowledge, and/or inefficiencies inherent to collaboration across various business entities involved in one given project.
ii) Time-consuming – or even occasionally unattainable – compliance requirements (e.g., traceability demonstration standards) generating significant complexity to retrieve and match key information from contracts, engineering specs, test procedures and results.
Generative AI in research is proving to be profoundly transformative. It can be used to research and analyze extensive amounts of documentation, both internal and external, such as engineering standards, design practices checklists, papers, and industry benchmarks, encompassing various formats, languages, and structures. It can identify and extract the relevant information and find the best way to display the requested information. It can also document user feedback, measuring engineers’ satisfaction and contributing to enhanced model performance.
GenAI can also support the reconciliation of information across the V-cycle, by extracting key elements, such as specifications and technical requirements, from technical documents (even when these are not properly referenced). Once retrieved, GenAI correlates the data to improve requirements traceability (e.g., high-level requirements with low-level specifications).
Finally, GenAI can accelerate the generation of test procedures and reports.
2. Smart search for technical documents
Engineering teams face the rising complexity of new or changing regulations, extended commercial and industrial ecosystems, technological constraints, customer expectations, and even their own organizational structure and business process management systems. As such, relevant information is often managed by different stakeholders, widely dispersed, and non-homogeneous in various regards (e.g., format, granularity, languages, etc.).
Engineering teams struggle to manage this complexity. Despite being a critical task, information management is highly time-consuming and potentially a source of new risks or missed opportunities.
GenAI can help human operators face these challenges, by leveraging its ability to research and analyze the extensive amounts of documentation required for maintenance and engineering, both internal and external, such as maintenance report, operating protocols.
Additionally, it can facilitate interactions between human operators and databases by writing and executing queries in natural language, which can prove decisive in accessing certain information.
3. Customer service efficiency
Within the consumer products industry, customer service faces several recurring challenges related to searching, summarizing, and classifying clients claims, as well as process logistics:
i) Customer service processes involve interacting with multiple IT systems and requires communication with various stakeholders to respond to customers’ requests, making it complex and tedious to gather relevant information.
ii) These processes are still largely manual, which is time-consuming and increases the risk of errors. As a result, end-to-end claims resolution can take months to complete, impacting both customer satisfaction and cash flow optimization.
To address these challenges, GenAI can be used at several levels:
- Data retrieval and synthesis: To search for and retrieve relevant information related to the claim from various data sources, such as invoices and delivery receipts and contracts, all of which exist in different formats.
- Proposition of insights and validation recommendations: Comparing collected information and received claims, GenAI can swiftly detect inconsistencies, highlight discrepancies, provide insights, assess whether the customer’s claim is well-founded, and make recommendations on potential outcomes to suggest to customers.
- Document processed claims: Capitalization is of the essence. Documenting processed claims, connecting the claim, its outcome, and the evidence used paves the way for easier information retrieval and decision making, should similar cases arise.
From patterns to trends: Key GenAI considerations
We expect the use of GenAI in all industries to scale up at an accelerated pace in the coming months. Below are some of the more exciting zones of development:
RAG: a powerful tool to improve accuracy and limit hallucinations
Retrieval-Augmented Generation (RAG) is an approach combining two basic capabilities of GenAI (information retrieval and content generation), both proven to be very efficient at producing highly accurate responses.
To put it simply, RAG essentially consists of restricting the search field in which a GenAI model will look for relevant information to answer users’ requests to a given, predefined, and limited set of documents. By doing so, this ensures the model will only look within a curated collection of information, the accuracy and quality of which can be guaranteed. This targeted retrieval is then used to generate a more precise and informative response.
RAG is particularly helpful in limiting or eliminating hallucinations, which are instances where the model might generate incorrect or nonsensical information. This is achieved by grounding responses in data coming from reliable and relevant documents. This approach is currently implemented in a vast range of use cases, in various industries where the reliability of responses is particularly important.
Three vectors for success for GenAI in R&D and operations
To lift the potential of Generative AI in Operations, like for any other digital innovation, companies must integrate the technology in their digitalization strategy, build scalable tools and upskill their staff on how to best use these AI tools in their data ecosystem. But GenAI also comes with its specificities. So, how do you avoid the pitfalls and find the steppingstones?
Final thoughts:
The GenAI landscape is evolving at a phenomenal pace – too great a pace for some. Barely had ChatGPT 3.5 dropped from media headlines when ChatGPT 4o was released and made free to the general public. With this in mind, it is vital that business leaders stay up to date on the technological roadmaps of software providers. This is the only way to know if solutions already exist or should be customized for a specific need. Additionally, be sure to systematically assess and then monitor the value creation of any custom solution developed in-house, all the while asking yourself one simple question: is it worth it?
“Capgemini partners with companies to transform and manage their business by unlocking the value of technology.
As a leading strategic partner to companies around the world, we have leveraged technology to enable business transformation for more than 50 years. We address the entire breadth of business needs, from strategy and design to managing operations. To do this, we draw on deep industry expertise and a command of the fast-evolving fields of cloud, data artificial intelligence, connectivity, software, digital engineering, and platforms.”
Please visit the firm link to site