Almost two centuries ago, Lewis Tappan and John M. Bradstreet illustrated the potential for turning data into a profitable product. At the time, businesses and merchants were expanding their operations and needed a reliable way to determine the creditworthiness of potential partners. Bankers and investors were eager for more consistent, objective information in this burgeoning economy to guide their lending and investment decisions. Tappan and Bradstreet established firms dedicated to collecting, analyzing, and selling data, along with the insights they derived from it. Their firms filled a critical gap in the market, eventually merging to form Dun & Bradstreet.
Fast-forward to today, when companies are often awash in data and trying to figure out if they can turn it into a business. The answer isn’t obvious. Building a data business is not for every institution, especially in a field where a few dominant players with massive advantages in data already exist. However, the potential rewards can be immense for companies that can unlock unique data, analytics, or organizational know-how to create a product that addresses an untapped market opportunity.
A European building-materials company identified a new-business opportunity with more than $500 million in enterprise value by turning an internal tool for tracking key performance indicators (KPIs) into a product it could sell externally. Similarly, a telecom company is on track to realize $200 million in new revenue in less than five years by using its data to build a digital lending business. And they’re not alone. McKinsey’s annual survey of business leaders on new-business building found that approximately 40 percent of them expect to create data, analytics, and AI-based businesses in the next five years—the highest of any new-business building category (Exhibit 1).
How do you know if building a data business can create value for your organization? In this article, we share why now is the right time to consider it, how to assess whether it’s a good fit, and what the critical considerations are for getting started.
Why now
While leaders have sized up data businesses for over a decade, evolving technology capabilities and greater adoption worldwide of AI and analytics have increased the feasibility of monetizing data today. Four technology shifts, in particular, have enabled companies to create new data products faster and less expensively than ever:
- Enhanced data-management efficiency: Companies can more efficiently process, manage, access, and reuse data in real time across different platforms thanks to greater sophistication of data tools and technologies. This efficiency is crucial for creating a scalable and sustainable data business.
- Generative AI (gen AI): A few years ago, converting unstructured data, such as text, images, and videos, into a standardized form so it could be accessed and analyzed was prohibitively expensive for most companies. Gen AI has made structuring such data more cost-effective, enabling broader use. Combined with the emergence of low-code and no-code analytics platforms that democratize AI and analytics, data businesses can now derive more value from their data.
- Increased access to real-world data: As Internet of Things (IoT) adoption accelerates, the costs and barriers associated with implementing sensor technology and capturing real-world data have significantly decreased. Companies can now gather real-world data more quickly and affordably and make it accessible to a broader range of applications.
- Growing use of internal data products: Industry leaders are increasingly treating data like a product internally so that a given data set can support many different use cases (see sidebar, “What is a data product?”). This “data packaging” gives them a head start in monetizing their data.
Additionally, we anticipate the thirst for data-driven decision making to intensify as leaders vie for their share of the up to $17.7 trillion in value potential from data and analytics. Add in another $2.6 trillion to $4.4 trillion from gen AI. This can create fertile ground for data and AI products. Consider Walmart Data Ventures, which launched a data solution to help suppliers better understand customers’ shopping behavior, among other insights. The company’s product, called Walmart Luminate, filled a gap in the market, enabling the company to achieve strong market adoption and 80 percent quarter-over-quarter growth during its first year.
Because data businesses often require strong value propositions and unique data advantages to win, we expect a small group of data businesses to emerge and dominate industry-specific markets over the next decade. Those who come later may find it difficult to catch up.
Assessing the opportunity and the right strategy
At its foundation, a data business must have access to a sizable amount of data (internal or external) or an approach to processing data and extrapolating business value from it that is unique enough to address an unmet market need. Demographic shopping data, for instance, may not be valuable today, given its ample availability from current market leaders in the space. However, data on real-time shopping preferences in niche market segments could be valuable to some companies as they localize market strategies.
In our experience, leaders can pursue three broad strategies for building such data sets, each with a different value proposition and critical success factors (Exhibit 2):
-
Create an industry standard, as Moody’s, S&P Global, and Fitch did for credit ratings. Typically, these data businesses start as data aggregators, assembling a massive amount of unique data. Some can reach a tipping point when a network effect scales the utility of their product until it eventually surpasses alternative offerings and becomes an industry standard. This can be a very effective strategy. Consider Reddit’s $60 million annual deal enabling Google to train its AI models on its data. But it’s also one of the most difficult business models to pursue. Once the market leaders become established as a de facto standard, it is increasingly difficult for new entrants to compete.
Succeeding here requires an asymmetric advantage in data access, a first-mover edge, or both. One financial-services firm has positioned itself to become the go-to choice for accurate pricing predictions in regions where pricing dynamics change quickly. The company has done this by collecting novel data from satellite images, listings, public filings, ads, direct calls, and business locations (sometimes dispatching individuals in person to capture specifics not available online) and analyzing it in a way that improves its predictive accuracy. It then offers customers an easy-to-use platform to access the resulting insights.
-
Harness insights from an engaged user base. With the appropriate data usage rights, organizations can turn data collected from an engaged user base into valuable insights for advertisers, suppliers, partners, and users. Benchmarks and behavioral data from digital interactions, for instance, can be sold “as is” via data marketplaces or combined with analytics and sold as insights directly to buyers. Companies can also use these insights to sell targeted ads on their digital channels.
This strategy depends heavily on the uniqueness of the data and the company’s ability to create a strong product value proposition for customers. The business case becomes more attractive if it can trigger a “flywheel effect” in which the sale of data products increases the sales or stickiness of core products. The financial-services firm paved the way for incremental revenue by stitching its popular data and analytics products into an intelligent workflow solution that automated a critical business process for its customers and accelerated their decision making. This integrated solution also boosted sales of the company’s other offerings because customers preferred to stay in its ecosystem for other data and analytics needs.
-
Turn sizable organizational know-how into a product. For example, knowledge and capabilities accumulated in building a tool to solve an internal business problem can sometimes evolve into a profitable offering. This was the strategy of the European building-materials company cited earlier that turned an internal tool for tracking efficiency into a software-as-a-service (SaaS) product. While leaders were initially concerned about cannibalizing the competitive advantage gained from this data, their analysis found that turning this data into a product would be more lucrative for the company than keeping the tool for itself.
This organizational know-how can also emerge as a company collects unique data as a by-product of its core operations. One company is transforming the way it operates and creating new data-driven revenue streams by adding IoT sensors to its assets and using the resulting insights to enhance its customers’ operations. For instance, with temperature and GPS data from the sensors, the company’s customers can make better routing decisions in transit for temperature-sensitive shipments.
Critical considerations for building an enduring data business
Leaders who identify a potential opportunity to monetize data should expect a three-to-five-year runway to achieve the economies of scale that are the foundation of a high-margin, enduring offering. (Launching a minimum viable product for market testing should occur within the first 12 to 18 months.)
Navigating this terrain successfully requires defining a strong customer value proposition, implementing an operating model and technology capabilities capable of scaling and sustaining the business, and addressing up front any data privacy and security concerns that might affect operations.
Defining a strong customer value proposition
We find there are generally two product attributes that can impact customer value proposition and adoption:
- The type of “intelligence” a data product offers. The classic DIKW framework—data, information, knowledge, and wisdom—offers one hierarchy for assessing the potential value and durability of an offering (Exhibit 3). Companies can create profitable products by selling volumes of raw data or information—basically data that has been contextualized in some way, such as purchasing habits extracted from sales data. However, the higher a data product ascends in this value chain, the greater its value for end users and the more difficult it is for competitors to replicate it, resulting in higher margins and customer retention over time.
- Product-delivery archetype. Raw data is usually delivered through a data platform such as a data marketplace. Other types of intelligence are offered through traditional insights platforms, such as an analytics tool, or intelligent applications integrated directly into an end user’s workflow. Here, the more integrated an offering is within an end user’s decision making and workflow, the greater its potential value to end users, the higher the margins, and the more likely it is that end users will come to view it as essential to their daily work, reducing customer attrition (Exhibit 4). Over time, as your customer base increases, a virtuous cycle is created in which data and feedback from a growing body of interactions further differentiate the offering and increase customer loyalty.
Early customer research and testing of a minimum viable product are essential in developing a new offering in order to avoid the trap of overestimating its potential. One common approach is recruiting a small group of target customers willing to be early adopters and to offer continuous feedback throughout the product build. A European pharmaceutical company ensured the successful launch of its highly anticipated new data product by initiating a consistent feedback loop with potential customers to validate every feature during development. This included frequent communication with customers and A/B-style prototype testing as it developed a minimum viable product. During development, subject-matter experts also validated the algorithmic outputs frequently, which was crucial to winning the trust of the pilot customers.
These feedback loops can be used throughout the product’s life cycle. While the financial-services firm’s initial offering was market leading, it realized through ongoing customer feedback that the value of its product increased with the timeliness of its insights. By creating a new workflow solution that delivered real-time intelligence at the point of decision making, the company could increase the value from its existing data assets fivefold to tenfold.
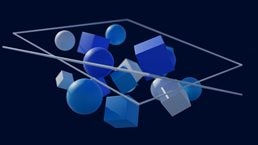
Adapting your operating model
One of the most common mistakes we have seen companies make in building a data business is neglecting to adapt their organizations and capabilities to effectively support the delivery of data products. Enterprises building data businesses need to orient their organizations around new profit-and-loss (P&L) expectations, new pricing and sales models, and investment in new technical skills:
- Incentivizing growth potential over short-term profits: Often, data products fail due to unreasonable performance expectations in the first year. As is the case with any start-up venture, during the first one and a half to two years, leaders should base incentives on KPIs that measure growth potential rather than short-term profitability. These KPIs typically include customer growth and retention, monthly recurring revenue (in the case of SaaS products), or lifetime value of a customer compared to the cost of customer acquisition (LTV versus CAC). One strategy to support this is separating the data business from the parent company through internal organizational and accounting controls or by creating a distinct legal entity. The European building-materials company, for instance, spun off its data business as a subsidiary, enabling the new entity to increase its autonomy and make decisions faster.
-
Adopting new sales and pricing models: On the sales side, most data businesses will need to hire new talent and upskill existing talent to explain and demonstrate a data product’s value—tailoring and delivering demos, engaging customers early with pilot programs, developing relationships with senior technology or data decision makers, supporting new pricing models (for example, freemium models), and helping clients understand deployment considerations. For instance, one large consumer data and research company seeded a digital go-to-market team to lead product-based sales efforts with free trials and targeted usage tiers as it built out its offering. Beyond initial sales, setting up an organization to assist clients in ensuring their customers’ success in using the product, as well as upskilling talent to provide ongoing client advice, can help improve the customer experience.
Pricing data products can be tricky, as the value of “better, faster decision making and workflows”—often fundamental benefits of this type of offering—can vary more than it does for traditional products. The utility of a data product isn’t always immediately apparent to customers, making it more challenging to convince them that these products soothe a distinct pain point, especially when other options are available. As a result, it is critical to invest in adequate pricing research to ascertain the product’s usefulness to the customer and what the customer is willing to pay for it. This research can also often guide a company on how best to align its offerings with customer pain points and position them against alternatives on the market.
- Investing in specialized technical skills: The goal is to support growth and sustainability of the data business and build out the company’s capacity to monetize data. The type of intelligence a data business delivers within the DIKW value chain will dictate the kind of technical talent a company needs. Leaders seeking to provide raw data will need to invest primarily in data engineers, while those seeking to provide end users with more sophisticated insights, such as knowledge or wisdom, will need to increase their bench of data scientists as well as their AI and machine learning engineers.
Modernizing your data technologies
Without a modern data architecture, it is tough for a data business to scale and sustain a leading customer experience. Depending on the starting point and complexity of data assets, a strong data foundation could take anywhere between six to 15 months to establish.
Some foundational technologies are table stakes that every data business will require (Exhibit 5). Additional investments will depend on the type of data and delivery method used. For instance, some data businesses, such as those seeking to provide customers with access to large volumes of data and information, typically provide a portal (commonly called a storefront) from which customers can search for and view details about the data sets.
Those companies that plan to offer an intelligence platform or develop a broad set of data products will need to embed strong MLOps and DataOps tooling, technologies, and practices into their platforms. These approaches enable companies to more rapidly, reliably, and cost-effectively deliver new AI capabilities as part of their offering, while effectively managing risk.
Managing data security, privacy, and intellectual property rights
Data security, privacy, and ownership are significant concerns for any leader. But the potential impact these risks can have on a data company’s business models and ability to expand raises the stakes significantly. As a result, leaders should ensure that their business, technology, cyber, and legal teams collaborate often and early on assessing the opportunities.
Following are four issues that will require early attention:
- Understanding the rights you—and others—have related to data: What are the sources of your data—first parties, vendors, and so on—and how was the data acquired? Are there limits to how you may use the data or concerns about whether it is derived from underlying data sets that have issues (for example, training data for generative AI that may be copyrighted material)? Data businesses should assess their data and closely follow the evolving conversation over data rights, particularly as innovative technology collides with, and spurs, these conversations.
- Developing consistent data privacy principles at inception: Identifying how the business will collect, use, retain, delete, and protect personal data before products launch can shield data businesses from potential setbacks and time-consuming hurdles when introducing new products and features, as well as uphold trust with customers.
- Examining and tracking local laws: Varying country, regional, and sector laws may influence how a data business collects, shares, processes, stores, secures, and manages data. Additionally, some jurisdictions have more clearly defined regulations than others, which leads to greater predictability. Leaders will need to consider their appetite for the uncertainty and risk of operating in areas where regulations are not so clearly defined.
- Prioritizing data governance and security: This is typically the “weakest link” that prevents data businesses from scaling. Data governance and security capabilities, such as quickly identifying and resolving data issues and effectively managing data access and entitlements, are foundational to delivering a quality product to a growing user base.
Building a valuable data set and associated insights can take time, giving those that move first a sizable advantage in seizing untapped market opportunities. But institutions that enter this market should have a unique data set that addresses an unmet customer need and the right capabilities to scale their product. Those who do may not only build a scalable and profitable business but also potentially create an enduring brand.
“Our firm is designed to operate as one—a single global partnership united by a strong set of values. We are equally committed to both sides of our mission: attracting and developing a talented and diverse group of colleagues and helping our clients create meaningful and lasting change.
From the C-suite to the front line, we partner with clients to help them innovate more sustainably, achieve lasting gains in performance, and build workforces that will thrive for this generation and the next.”
Please visit the firm link to site