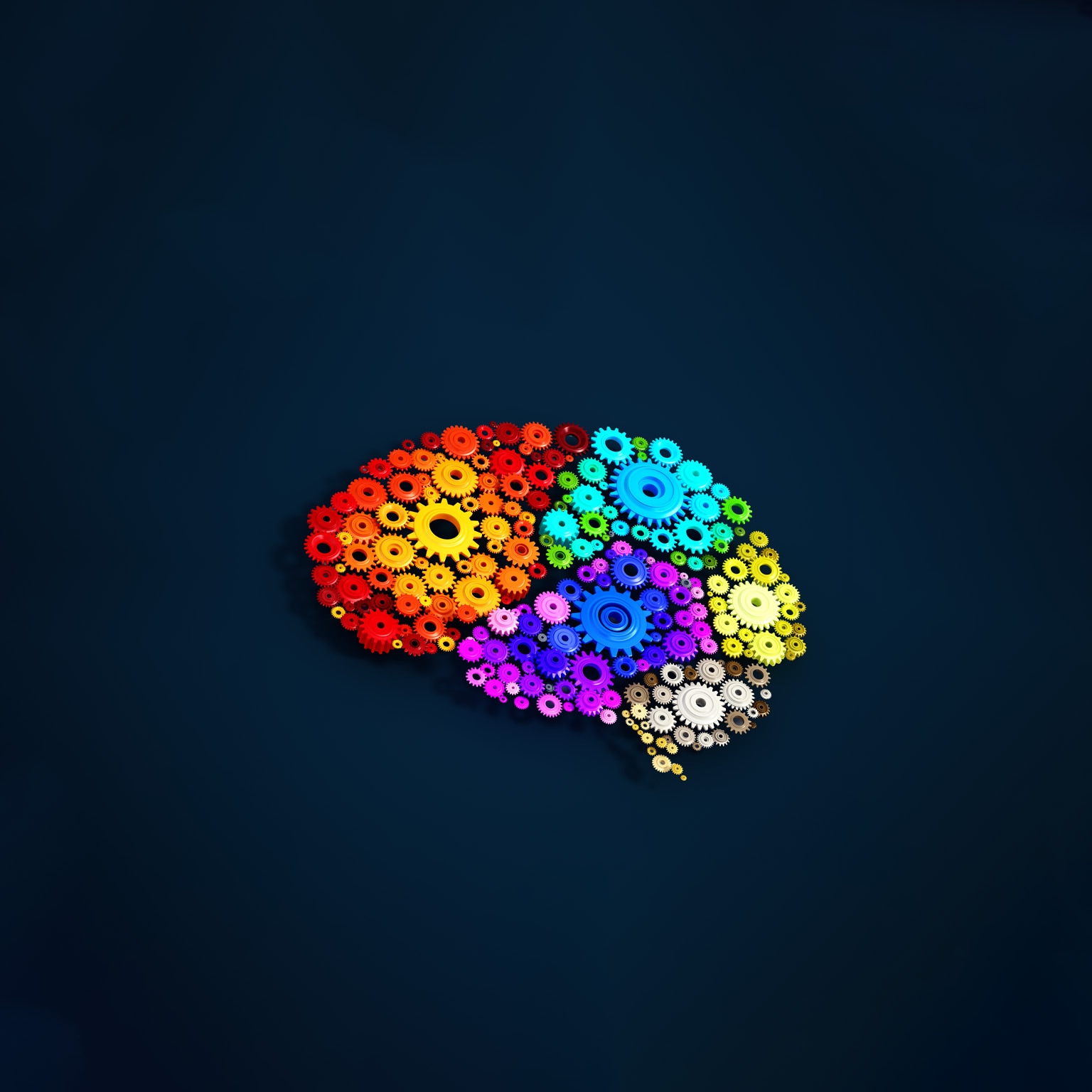
While AI continues to influence the way we work in exciting new ways, it is crucial for organizations to apply guardrails to keep it safe. On this episode of The McKinsey Podcast, McKinsey senior partners Alexander Sukharevsky and Lareina Yee dig into new research on AI adoption, with editorial director Roberta Fusaro.
In our second segment, how do you muster the courage to talk about something uncomfortable at work? Senior partner Sherina Ebrahim has two tips.
This transcript has been edited for clarity and length.
The McKinsey Podcast is cohosted by Roberta Fusaro and Lucia Rahilly.
AI’s time to shine
Roberta Fusaro: We’re here to discuss the latest McKinsey report on the state of AI, a technology evolving at an exponential rate. When it comes to gen AI, which is just one type of AI, our latest results show that 65 percent of our respondents reported their organizations regularly use it. This is double the percentage from our previous survey, which we conducted less than 12 months ago. Why is this new number important?
Lareina Yee: This number represents optimism. Even though we have a long way to go, the number shows that people are moving from curiosity to integrating it into their businesses.
What’s also important to note is that the report is not just looking at generative AI. It’s looking at AI overall. This has been a trend 40 years in the making. One of the things we’re seeing is that all of this excitement about generative AI is providing oxygen and daylight to the broader set of capabilities that can really help companies advance.
Alexander Sukharevsky: Yes, generative AI allows us to democratize about a 40-year AI journey because it is so in our faces that we can really see and feel what it is. We’re able to interact with it. Our clients’ kids are interacting with this technology, and it’s getting discussed over dinners. So something that used to be a niche market is suddenly part of the mainstream.
On the other hand, when 75 percent of respondents say that generative AI is used in their organization, the next question should be, what exactly are they using it for? Are they using it for experimentation, familiarizing themselves with the technology, or are they actually trying to unlock true business value?
Partnering for AI success
Roberta Fusaro: Staying with generative AI, Alexander, about half the respondents in our research say that they are using readily available gen AI models rather than building their own. What are the pros and cons of doing that?
Alexander Sukharevsky: One important fact to bear in mind if you step back is that only 11 percent of AI models end up in production, meaning they become day-to-day true business tools to unlock value. If we consider some of the cost and risk of generative AI, this number is close to a single digit when we’re speaking about the traditional enterprise and not just tech companies. Therefore, it’s important to recognize that the model itself makes up only 15 percent of the success.
Now we are moving into paradigm of not just “build versus buy” but “build, partner, and buy.” There are certain open-source models with amazing community support that organizations can customize to their needs. There are some proprietary models with very high investment behind them that organizations cannot develop themselves. And there are some models that organizations will develop in partnership with third parties.
At the end of the day, the enterprise of the future will have a spine brain of dozens of foundational models. Some of them will be proprietary that you buy. Some of them will be those that you develop on your own. And some of them will be open source. So the answer to this question [to buy or build] depends on the client.
Lareina Yee: This is an important topic because it is a classic question to wonder about “buy versus build” in technology. But Alexander and I have been working on this in the deployment, and I think we’re in a different paradigm. Being part of a partnership is a really important point that Alexander is raising. It is hard to build all of this on your own. It is also not feasible to buy all of this on your own. What you’re finding is that you have to partner across the stack. That’s kind of a traditional tech term. What that means is you’re going to partner with large language model providers. There are lots of choices.
So the point of all this is to drive and unlock some business value that you weren’t able to access before. We’re seeing a lot of companies build a constellation of partnerships in order to deliver the promise of gen AI solutions.
Alexander Sukharevsky: But to power the models, you also need the compute power. You will need certain partners that will allow you to get this compute power. And even if you are the most powerful and well-resourced organization in the world, you cannot make it yourself.
Lareina Yee: The number-one question to ask yourself is, “What is the business use case that I’m trying to achieve?” And based off that, “What are the sets of providers that are going to help me most?” That might be a combination of a very large language model provider that’s very enterprise focused. It could be someone who has stronger strengths in video but is doing something around text.
And so, even though this is a fast space, I think it always comes back to, “What is the business objective? What’s the people objective?” And then, just being far more open in your mindset of how you bring in different technology providers and different combinations of partners to achieve that quickly.
The future is still human
Roberta Fusaro: Talent is a huge question and issue for everyone. I’m curious about what the research showed, or if there are different talent imperatives for executives who are trying to get ahead on gen AI.
Lareina Yee: Talent is always top of mind. You have to get really practical and match the talent to, for example, a gen AI, AI, or machine learning solution. Those have different use cases. But in all cases, there are sets of capabilities that are going to be really important for your team. And the number-one thing we see in the report, and we see in our own experiences with clients, is data capabilities. So how you think about data and the type of talent you have is important. That’s just one example of the type of talent you need to implement these solutions.
When we’re asked, “What’s going on with talent,” the question is typically more about jobs gained/jobs lost. And that’s more of an economic question. And for that, we know these technologies do change the fabric of jobs. But one of the optimistic things we see is they also create new jobs. So what we see in terms of talent is there are many different aspects of it. There’s the talent and capabilities you’re going to need as a company to develop and scale these solutions. There’s also an overall talent question in terms of how they change the fabric of jobs.
Alexander Sukharevsky: One of Lareina’s favorite quotes is, “On every dollar of technology we need to invest three to five in human beings,” because human beings are very expensive and difficult to change.
So the real questions are: “Beyond having an amazing technology department, who’s able to help you to operate and build the tools? How do you convince the rest of the organization to really use these tools, to embrace them, to manage risk vis-à-vis any other third party?” These are the difficult questions where you take colleagues who are coming completely outside of technology to learn technology and to trust technology. That’s quite a journey, be it around change management or be it around capabilities.
Lareina Yee: We spend so much time on the technology. But in fact, that’s the easy part. The harder part is the human change. And we also sometimes lose the plot here. The purpose is not generative AI as a technology. The purpose is generative AI as a tool to help humanity. People are at the center of this. And that change is hard. There’s that level of micro change.
The purpose is not generative AI as a technology. The purpose is generative AI as a tool to help humanity. People are at the center of this. And that change is hard.
Lareina Yee
There’s also the macro change, “Do I trust how I interact with a machine differently? How do I feel about potentially leaving actions to a machine?” We’re starting to see the rise of agentic capabilities, which is where these systems can take an action. There are a whole host of questions, and us getting more comfortable with that is a journey, and changing the fundamental business processes that we use—that’s the hard stuff.
Use cases and applications
Roberta Fusaro: I’m curious if in the new research we’re seeing different sorts of applications of generative AI. Are there parts of the organization where we’re seeing it more or less?
Lareina Yee: Looking at the report, the most common domains we see are marketing and sales. We also see enormous amounts of work in product development and software engineering. These arenas are expected because these are where the types of knowledge work are most applicable to the capabilities of the technology today, especially when the vast majority of what we’re looking at is more the summarization and concision of text.
We also see a difference by industry. It’s not surprising that we see the technology sector, the energy sector, and financial services sector being the sectors that are probably the furthest along in experimenting and beginning to deploy these capabilities at scale.
Alexander Sukharevsky: The way to look at this is that generative AI, essentially, is the most convenient human interface to apply other AI techniques. And therefore, it’s all about interface; be it interface with a database, be it interface with other algorithms, be it even interface between different generative AI applications.
I think if you fast-forward, to Lareina’s point, you will see more and more autonomous virtual agents communicating with each other to solve different tasks under strict human supervision to properly manage risk as well as to ensure that the quality of deliverables is going to be up to the standards that we’re looking for. Therefore, while currently we’re seeing mostly interaction that is human versus machine, as we develop, we’re going to see more and more machine-to-machine interactions to solve different tasks. Now we’re not talking about superintelligence or AGI [artificial general intelligence]; we are years away from that moment. At the same time, we’ll see very sophisticated, very niche assistants that will help us to do our job better, faster, and more precisely.
Limiting the risks of AI
Roberta Fusaro: There’s lots of opportunity, clearly, given our conversation so far. But according to our report, two of the top risks most often cited by organizations when it comes to their use of gen AI are inaccuracy and IP [intellectual property] infringement. Have organizations started to mitigate some of these risks? And if so, how?
Lareina Yee: So when we look at the risks, there are a lot of risks. And one of the things both Alexander and I remind our clients about is that these are the early innings of the technology. Inaccuracy is one of the risks that people are most concerned about, but there’s also intellectual property infringement, cybersecurity, individual privacy, regulatory compliance, explainability, fairness, and amplification of bias.
For those that are developing these large language models, they are working really quickly on many of these risks. Explainability is another one. There’s also the reduction of hallucinations, which is something that you’ve seen has gotten better over the course of the year.
It’s not down to zero, but there’s been a lot of work on the provider side to make sure that it’s better. And that’s going to improve the inaccuracy issue. On the other side of this is companies’ implementation. How they develop, train, and test these systems is incredibly important before they let them out.
Alexander Sukharevsky: The most important part to really understand is, “What are the risks?” Because if you look at our report, the majority of respondents believe there are risks, yet they cannot articulate what these risks are. There are ways of solving these risks. Number one is clearly having a human in the loop. And that’s why I don’t like to speak about artificial intelligence. I rather prefer “hybrid intelligence,” where we bring the best of humans and machines working together to overcome the challenges and the risks and unlock the opportunities.
The most important part to really understand is, ‘What are the risks?’ Because if you look at our report, the majority of respondents believe there are risks, yet they cannot articulate what these risks are.
Alexander Sukharevsky
On the other hand, should we think that technology can’t help us solve some of these issues? For example, as to IP or traceability, you could apply technology to track the IP, to protect IP. At the same time, while we believe that we all focus on very short-term risks, I do believe that we, as humanity, should step back and think, “What’s the bigger picture? What does this thing do for us, for future generations? Where should or shouldn’t we apply it—be it from a humanitarian point of view, a social point of view, or an environmental point of view? What type of future are we shaping by applying AI as a technology?” And those are significantly bigger questions that we should spend more time with, within the boardrooms as well as the machine rooms, to ensure that we understand exactly where we are heading.
Lareina Yee: I think there are some incredibly long-term questions, Alexander, and some of them are very philosophical in terms of our relationship with machines. I also think one of them is the human capacity for adaptability and creativity. And let me take a simple example, something that any parent, any student, any teacher might relate to, which is the very practical concern of plagiarism. This has come up a lot—that concern that students might use ChatGPT or Claude to plagiarize. That’s a real concern and not a risk of the system. That’s actually in the usage.
There is an incredibly practical hack that some teachers are using, which is that the exams are written in the classroom. We have this old technology called handwriting and pencils and paper that we can use to show that we have mastered the information. And it’s a very simple example, but what it shows is that there are some incredibly important ethical, very large questions that introducing these capabilities into our day-to-day lives brings.
Responsible AI governance
Roberta Fusaro: Lareina, you’ve written a bit in the report about AI governance, and that seems related here to the risks and making sure that we don’t go too far. How can companies begin to put some teeth into their AI governance?
Lareina Yee: As Alexander and I talk to companies, we start by saying, “Responsible AI starts day one.” So in a traditional world and with previous generations, the way that we may have thought of this is you develop a solution, and then you make sure to catch the risks and have a compliance function.
We absolutely need all that strength in our compliance, but we also have to move upstream and bring responsible AI on day one. So what does that mean? It means at a governance level, you’ve got someone with responsible AI capability and expertise that’s at the table making the decisions. That might be at your C-suite level, having someone help have that discussion.
It also means that, as you’re developing these solutions, you are testing and integrating how you develop these solutions to ward against things like bias and inaccuracy. So how we think about responsible AI isn’t a moment. It’s embedded in the way in which we develop our business plans, in the way in which we build, configure, and test the solutions, and the way in which we implement it and continue to get feedback, and the way we have strong compliance on the back end if there was a mistake made.
Steps for realizing value
Roberta Fusaro: What are some first steps for organizations that want to make sure that they’re starting to realize value from their investments in gen AI?
Lareina Yee: I think the first step starts with having the success metrics. What are you trying to achieve with this? Deploying generative AI just to say you’ve done it, just to create a conceptual demo or gizmo, that’s not going to lead to business value. At the very onset, it’s important to say, “What are the success metrics? What will I see quarter over quarter?” And then, “How are we doing against that?” So that might be that you expect 20 percent more productivity, and you’re going to use that extra capacity to reach more customers.
Alexander Sukharevsky: This step-back moment is extremely important. And once you identify what you’re looking for, you should go back to the recipe that we discussed before in terms of, “What does it take to scale and embed AI within the organization?”
Lareina Yee: Alexander, I love your point on scale because sometimes people ask, “What does it mean to scale?” If you only have ten engineers using the solution, that’s not scale. Scale is when you have the vast majority of the engineers using the solution and actually showing results out of it.
Arguably the harder and the longer step is the adoption curve of users, and everybody using it, and changing work. That takes real time. So you may have the solution out in 12 weeks, but do you have the adoption and the usage out in 12 weeks? No. You must continue to work on that quarter over quarter, where over the course of a year or 18 months, you’ve gotten the type of business results that you aspire to have.
The future is bright
Roberta Fusaro: What are your final thoughts about where we’re heading with gen AI?
Lareina Yee: The technology and its capabilities are unbelievably exciting. In order to capture it, we need to bring back that sense of pragmatic decision making. What are the cases that are going to make a difference in our business? How do we start to invest fully? How do we invest in these cases? How do we bring them to life? And how do we create that value for our businesses? I think we’re headed into an era of important pragmatism.
Alexander Sukharevsky: I agree with Lareina with the caveat that we are still at the pre-awareness phase because the technology is so new. In less than a year, ten million developers got access to these tools. So what we are seeing now is just the beginning, and I believe it’s therefore the era of creativity and imagination.
Though we kind of understand what it might do, we haven’t had enough time to figure out how to reinvent our business models and the way we work today. Together with the pragmatism that Lareina was talking about, and the imagination I mentioned, I think in the next 12 to 18 months we will see breakthrough pragmatic solutions, where you apply technology not just to entertain yourself but to unlock true value, be it for business or more important, for humanity.
What to do when you’re not being heard
Lucia Rahilly: Next up, McKinsey senior partner Sherina Ebrahim shares two tips to help anyone confronting their manager’s irritating behavior.
Sherina Ebrahim: The first time I returned from parental leave, I was a manager, and I had come back to work part time. Back then, working part time was a well-established policy, but it was not as widespread as it is today, particularly for the manager role.
When I came back, I took off one day a week. The first week, the partner that I worked with had a meeting scheduled for my day off. I did the meeting anyway. The second week, the same thing happened, and I did the meeting. Then the third week, the same thing happened: we had an internal team meeting scheduled, and again I didn’t say anything. But at this point, I thought I needed to say something.
So I said to the partner, “You know, I am working part time and tomorrow is my day off, and we’ve now scheduled, for the third time, a meeting on my day off.” And honestly, the partner was mortified. He had just completely forgot. He fully apologized and we changed the meeting, moved on, and the rest of the engagement was perfectly fine in terms of how we made it work.
The learning for me from that was two things. The first was assume positive intent. It really was one of those, “I’m not used to it, just moving from one thing to another, just wasn’t thinking about it” type of things. The second is stand up for yourself. When you see something that doesn’t quite work for you, at least bring it up and have a conversation.
I think that helped me for the rest of my career because of course, things aren’t always perfect. There are going to be times when you’re working part time, and if you don’t actually have the conversation and engage, you don’t know what your manager is thinking, they don’t know what you are thinking, and it actually doesn’t lead to a positive outcome.
“Our firm is designed to operate as one—a single global partnership united by a strong set of values. We are equally committed to both sides of our mission: attracting and developing a talented and diverse group of colleagues and helping our clients create meaningful and lasting change.
From the C-suite to the front line, we partner with clients to help them innovate more sustainably, achieve lasting gains in performance, and build workforces that will thrive for this generation and the next.”
Please visit the firm link to site