As climate change accelerates and wreaks more and more havoc across the globe, a crucial question is how we can increase the share of power produced by renewables while simultaneously reducing demands on the electrical grid. Policymakers have an important role to play in this transition, but businesses arguably have a bigger one.
In 2022, the U.S. produced 4.24 trillion kilowatt hours of electricity, which resulted in $488 billion in revenue. The energy sector is one of the largest and most important parts of our economy, and it is also a prominent focus of research, teaching, and student pathways at Tuck.
The Revers Center for Energy, Sustainability and Innovation serves as a hub for much of this activity, with a mission to inspire and shape tomorrow’s leaders in energy while engaging today’s energy community. The center is led by Erin Mansur, the Revers Professor of Business Administration, whose research focuses on topics of energy economics, environmental economics, and industrial organization. He also teaches classes on energy economics.
Beyond the Revers Center, energy is a theme that appears in the research of faculty from numerous areas. Three new working papers illustrate the rigor and relevance of Tuck faculty on issues of energy and climate change, showing the breadth of Tuck research and its practical implications for reducing greenhouse gas emissions.
Using Pricing and Automation to Reduce Electricity Demand
Praveen K. Kopalle, the Signal Companies’ Professor of Management, is a leading expert on pricing and marketing analytics. In two recent papers, he explores how a dynamic pricing strategy, combined with automation of home appliances, can reduce electricity demand during peak hours and shift some of that demand to off-peak hours. The total effect is to greatly reduce greenhouse gas emissions from electricity generation.
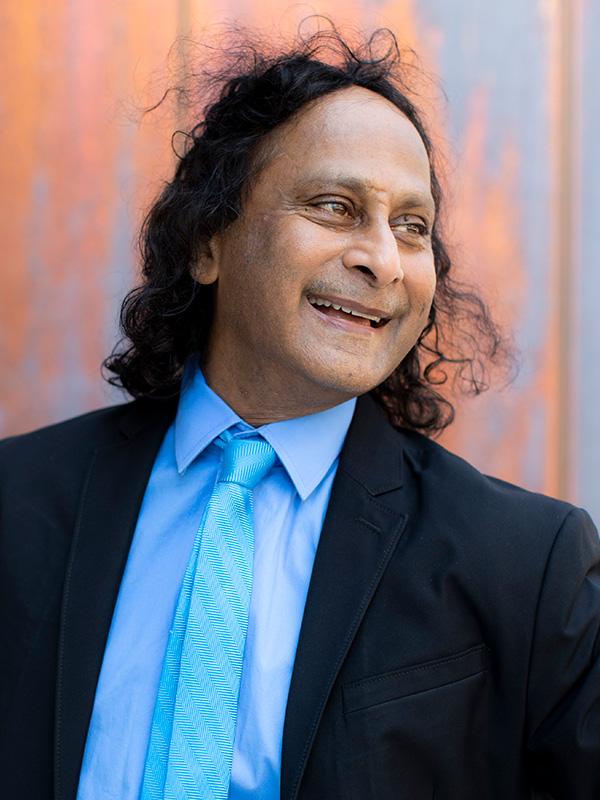
Kopalle’s first paper (forthcoming in Management Science) in this series is “Field Experimental Evidence on the Effect of Pricing on Residential Electricity Conservation,” written with Jesse Burkhardt of Colorado State and Kenneth Gillingham of Yale. In it, they analyze a two-year field experiment involving 280 Texas households, where households in the treatment condition were notified in advance about a critical peak period when electricity prices would spike, due to high temperatures and high demand for air conditioning. In response to this information, households reduced their electricity use by 14 percent and emissions by 16 percent during 27 of the hottest days over two summers. Because these homes were equipped with appliance-level electricity meters, the authors could see that 74 percent of the critical peak response was due to a reduced use of air conditioning.
In a separate pilot program, consumers responded to reduced nighttime electricity prices by shifting their electric vehicle charging to nighttime hours, when electricity demand is the lowest (and wind generation is highest). Kopalle and his coauthors suggest these dynamic pricing tools could be linked to appliances and EVs, so they automatically reduce their electricity usage during peak times and shift some usage to off-peak hours.
“These results provide guidance for business leaders and policymakers by suggesting that pricing and automation measures hold promise for fostering pro-social behavior and aiding the shift to renewable energy,” they write.
Kopalle builds on these findings in his second paper (forthcoming in the Journal of the Academy of Marketing Science), written with Burkhardt, Gillingham, and Tuck colleagues Lauren Grewal and Nailya Ordabayeva. In “Delivering Affordable Clean Energy to Consumers,” the researchers develop a marketing-centric framework that informs a system-wide solution for the adoption of clean energy by consumers. More specifically, they study how to leverage marketing principles and automation to not only get consumers to buy-in to clean energy, but also to reduce the need for fossil-fuel-based electricity generation for those inevitable gaps in renewable generation.
A solution for delivering clean electricity to residential customers lies in jointly leveraging multiple components of the 4Ps—[price, product, place, and promotion]—which could be coordinated by the local utility or a third party.
— Praveen Kopalle, the Signal Companies’ Professor of Management
The authors make use of the “4P” marketing mix variables—price, product, place, and promotion—to better understand consumers’ motivations and barriers relative to clean energy. They explore these variables in a consumer survey, field experiment, and decarbonization simulation. Their field experiment confirmed the positive effect of the critical peak power pricing strategy, and it added an element of automation, using NEST thermostats that can be synchronized with the utility company to automatically reduce air conditioner usage during peak power periods. Their survey showed that consumers are willing to absorb a small price differential for an automated solution. And their decarbonization simulation revealed that the dynamic pricing, combined with automation, could reduce the need for energy capacity by 53 percent. This, in turn, means that the costs of energy storage (i.e., batteries) could potentially be absorbed by local municipalities and states that are moving to a 100 percent carbon-free environment.
“We thus propose that a solution for delivering clean electricity to residential customers lies in jointly leveraging multiple components of the 4Ps,” they offer, “which could be coordinated by the local utility or a third party.”
Using Optimization to Decide Which Power Plants to Turn On
The advent of renewable energy sources such as wind and solar power has been wonderful for reducing our reliance on fossil fuel and cutting emissions of greenhouses gases. But renewable sources make it tricky for utilities to know which of their non-renewable powerplants to use on any given day.
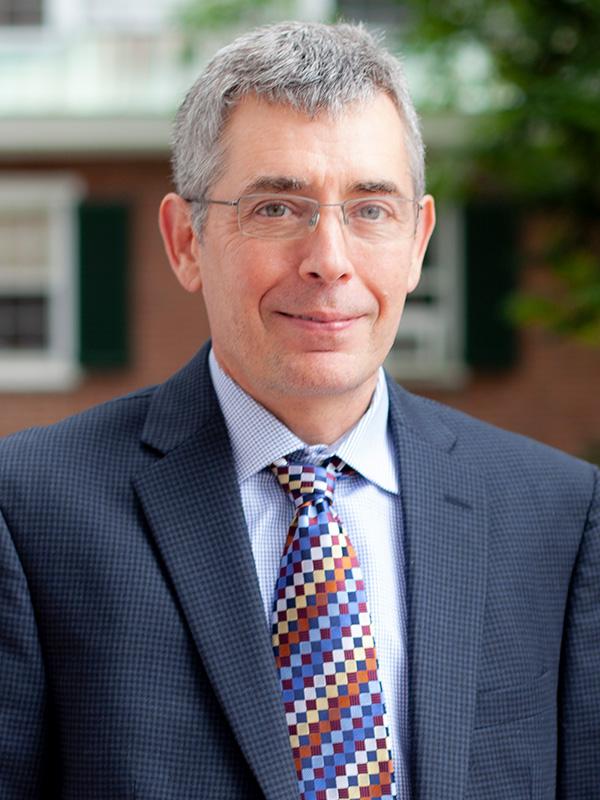
The advent of renewable energy sources such as wind and solar power has been wonderful for reducing our reliance on fossil fuel and cutting emissions of greenhouses gases. But renewable sources make it tricky for utilities to know which of their non-renewable powerplants to use on any given day.
On an average sunny day, solar panels are sending significant power into the grid from late morning to late afternoon, and during this time, the need for fossil-fuel-based powerplants is diminished. However, if a thunderstorm rolls in and the sun stops shining, those traditional powerplants become more crucial to supplying the base load of electricity. Every day, Duke Energy, which owns 130 powerplants in the Southeast, must look at the weather forecast and decide which of its powerplants to turn on, and when to do so. This is referred to as the “unit commitment problem” in the utility industry. This is complicated for many reasons, one of which is that some of these powerplants take hours to turn on and off. Duke’s current process, therefore, is not only complicated; it’s also potentially fraught with expensive wrong guesses.
In new research, Jim Smith, the Jack Byrne Distinguished Professor in Decision Science at Tuck, aims to make this operations decision-making process easier and smarter. Smith is partnering with Duke Energy and his former Duke University colleague David Brown on the research, which is supported by a grant from the Department of Energy’s Advanced Research Projects Agency–Energy (ARPA-E) that addresses uncertainty in the electric utility business.
As Smith explains, “our research question is can we just put uncertainty into the whole planning process, as opposed to an ad-hoc deterministic plan that’s adjusted hour by hour?” Their overall approach is to create a dynamic contingent plan that determines powerplant usage in every possible state of the environment. In essence, their optimization model crunches the numbers—price, demand, capacity, etc.—and calculates a vast array of operational possibilities, anyone of which can be deployed given the realities of the day.
After testing their model on demand scenarios from the past and altering those scenarios for a future that might contain a carbon tax, the model showed a significant improvement over the current practice at Duke Energy.
“On average,” Smith says, “The current practice at utilities like Duke is approximately 2.8 percent suboptimal. In the future world with a carbon tax, they are 6 percent suboptimal, and 8 percent suboptimal without a carbon tax.” In contrast, Smith and Brown’s model is no more than 0.2 or 0.3 percent suboptimal. “Our model can handle any scenario, while their performance gets worse with more uncertainty,” he says. In terms of dollars and cents, Smith and Brown’s model saves a utility the size of Duke Energy up to $1 million on a high load day by smart management of fossil-fuel powerplants and can reduce emissions at the same time.
Smith sums it up by invoking a famous quote from Mike Tyson: “Everyone has a plan until they get punched in the mouth.” To avoid that trap, Smith’s model doesn’t “commit” to a specific plan—the title of the paper, tellingly, is “Unit Commitment without Commitment.”
“Our planning process is about expecting the punches to come, even if you don’t know precisely when or from where,” Smith says. “And then, staying light on your feet and rolling with the punches when they do come.”
Our planning process is about expecting the punches to come, even if you don’t know precisely when or from where. And then, staying light on your feet and rolling with the punches when they do come.
— Jim Smith, the Jack Byrne Distinguished Professor in Decision Science
“On average,” Smith says, “The current practice at utilities like Duke is approximately 2.8 percent suboptimal. In the future world with a carbon tax, they are 6 percent suboptimal, and 8 percent suboptimal without a carbon tax.” In contrast, Smith and Brown’s model is no more than 0.2 or 0.3 percent suboptimal. “Our model can handle any scenario, while their performance gets worse with more uncertainty,” he says. In terms of dollars and cents, Smith and Brown’s model saves a utility the size of Duke Energy up to $1 million on a high load day by smart management of fossil-fuel powerplants and can reduce emissions at the same time.
Smith sums it up with a powerful analogy: “The old Duke plan is like driving directions on a paper map,” he says. “Our plan is like Google or Waze, which changes the route as you drive, to account for traffic.”
Tuck School of Business
Please visit the firm link to site