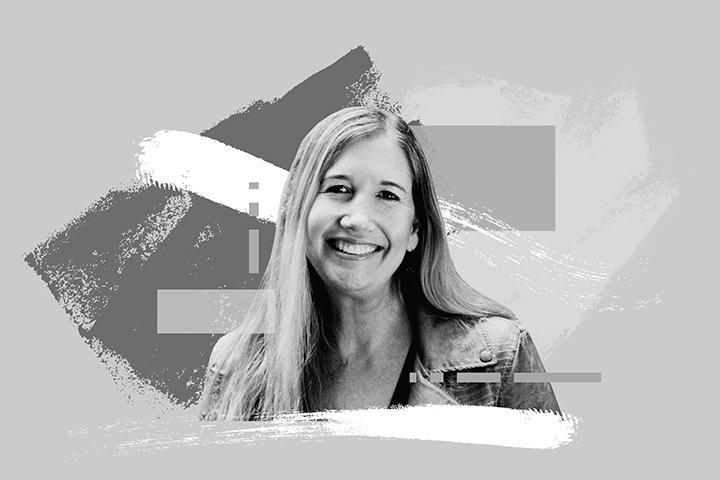
JAIME TEEVAN: Thanks, Molly. It’s a pleasure to join.
MOLLY WOOD: So, you have the most fantastic-sounding job at Microsoft. Can you tell us a little bit about it? What was Microsoft CEO Satya Nadella’s initial vision for your role? And then what’s your day-to-day like?
JAIME TEEVAN: Yeah, I do have the most fantastic job, so you are right. [Laughs] My job is to drive research-backed innovation in our core products. And, yeah, I had no idea how exciting this would be. You know, Satya asked me to take on this role as Chief Scientist, thinking about innovation in our products, because he knew that a big disruption was coming and that it was necessary to really think differently, even with all our background, and even with all of our history, to think differently about how computers will help people get things done.
MOLLY WOOD: Yeah. Before we dig into a little bit more about using AI effectively, I wonder, tell us from that perch about some of the insights that you had that sort of said, this is how we can actually use AI to create a connective tissue that would eventually become Copilot to, you know, add these huge productivity gains.
JAIME TEEVAN: A lot of times we think about work as this thing that requires a lot of focus and you have to sit down and the only way you can get anything done is if you have an hour to really think and stew on things, but that’s just not how the real world works. You know, we all have meetings and emails and things that are coming in. I have four kids that are bugging me and interrupting me all the time. There’s just a lot going on. And, actually, people are well set up to do that. We are used to operating in a complex environment where there’s lots of things happening and lots of things going on. But it does mean we need to be very intentional about how we transition our attention. So I did a lot of work thinking about, how do we use AI intentionally to transition people’s attention and help set people up to be really successful. And that is at the core of Copilot.
MOLLY WOOD: So, one of the things that you’ve said about this new phase of AI advancement is that it connects us to one of the most ancient technologies, which is natural language. Talk a little bit more about what you mean by that.
JAIME TEEVAN: We’ve all been talking since we were little children, and people have been using natural language to communicate for hundreds of thousands of years. The big change in the past couple of years is that now we’re using it to communicate with our computers as well. And that’s really different. It’s a really big transition to think about not going to an application and having to translate whatever you want to get done with Excel or Word or PowerPoint into a bunch of button clicks and actions that you take, but rather being able to start directly with your intent, rather than saying like, okay, I want to think about my budget for the coming quarter. You used to have to open up Excel and think about the pivot table that you’re going to create. Now you can actually directly ask questions and say, what does my budget look like? How should I be thinking about my various different expenses and things like that over that period of time. So that ability to communicate in natural language is foundationally different. My background is actually in search, and search is a really great example of probably the first AI application that people used at scale, and in many ways search is where you first practiced expressing your intent to a computer. When you go to a search engine with a query you’re saying something about what you’re looking for, and now we have an opportunity to do that in a richer way, in a way that maintains context over an entire conversation or across multiple conversations, rather than thinking about how I’m going to express my intent in just one or two keywords.
MOLLY WOOD: Yeah, so previously we had this simple, kind of query-based search world. Now, maybe with large language model–based AI, we can use our natural conversation patterns and communication.
JAIME TEEVAN: Well, so we actually know a lot about natural language, which is cool. Like, we know a lot about how humans communicate to each other. We know, for example, that a large part of any conversation is comprised of grounding. It’s that back-and-forth conversation that we have to arrive at a shared understanding. That’s an important part of conversation. And so the fact that language models can hold an entire conversation in their awareness and operate over that is a really big difference compared to search. Each time you issue a new query in a search engine, for example, you get an entirely new set of results. Language models have all of that context that they use.
MOLLY WOOD: Right. And talk about how hard it is to express that to people. I think people are starting to understand that interaction, and that obviously we’re a long way from Boolean operators, which I remember from early search days, but that that idea of context is what really changes the game and allows you to refine and dig deeper and get to a really optimal outcome.
JAIME TEEVAN: Yeah, absolutely. So, kind of naively, when we’re thinking about working with AI, you just think of like, oh, there’s this really amazing model that underlies the whole interaction. But like exactly as you said, the context that is used to ground that conversation is at least as important, and that context is comprised of the prompt that a person uses with the model—so that’s like the instructions that you gave. You might say, write this email for me or help me understand this document. But then it’s also comprised of a bunch of other pieces, which includes, for example, the whole conversation that you’ve been having. Each time you have an interaction with a model, what it does is it basically takes the conversation up to that point and uses that as context in your prompt. It can also include things like the context of the document you’re reading, or the emails that you’ve gotten, or even more cool is when it uses all of the context of the documents that you have, and the transcripts from the meetings you’ve been at, and can operate over all of that information. You feel like it’s just that simple little instruction, but there’s all of this rich stuff that goes into it.
MOLLY WOOD: Right. Well, and then let’s talk a little more about prompts, because there has been this sort of question of creating the right prompt, and you’ve said, listen, you also need to outsource that to AI. Let it help you create the prompt that you do not yet know you need.
JAIME TEEVAN: One of the things that I actually think people make a mistake with sometimes when they use AI is they go in and they think they have to know everything, like, oh, I have to tell the model what to do. I have to know exactly what I want. I have to figure this all out. And it’s like scary and overwhelming, actually. And the truth is, one of the model’s greatest skills is this ability to help make sense of things and help do some of that planning and reasoning with you. And so actually, if you want to figure out how to prompt a model well, one great way is just ask the model to help you. Be like, I’m trying to figure this out. How should I turn this into a good prompt to get something done?
MOLLY WOOD: I mean, again, I think that the human need, especially in a business context, to seem like you know everything feels like it gets in the way. Like, you’re really just sort of saying it’s okay to approach this with a child-like posture and say, I don’t know what I don’t know, can you help me?
JAIME TEEVAN: Yep, and the good news is the model doesn’t think you’re stupid, so you can ask really dumb questions. [Laughs]
MOLLY WOOD: I mean, on the same note, there’s also been a lot of conversation about whether it pays to be polite in your interactions with AI. And you told the Wall Street Journal, like, yes—I’m quoting—if you speak to the model rudely, you can expect it to be difficult with you too. Can you unpack that for us?
JAIME TEEVAN: Language models are trained on natural language, right? What we do is we take large corpora of content and, essentially, we mask out one word at a time and then try to guess what that word is. It’s like a giant game of Mad Libs, basically, that the model is playing. And then when it gets it right, you give it a reward. And when it gets it wrong, you say, oh, go try again and adjust your weights and do a better job. And so in that way language models have essentially just done a really good job of predicting and guessing language. And so, if you start in a formal tone, it will mimic that formal tone. If you start in a polite tone, it will follow and be polite. And if you’re argumentative and rude, it will be rude back as well. So it pays to be polite to the model. But it also is useful to help the model understand what you’re looking for. One great trick for getting a good response is to give it some examples. So, like, if I’m trying to think of a title for a document I’m writing, I might first come up with some on my own and say, come up with a title like one of these two titles, and then have it go from there.
MOLLY WOOD: So we’ve started to get a handle on the ways that AI can help us spend less time on these mundane, lower-value tasks. But you are also urging people to think beyond those, right? Tell us more about that.
JAIME TEEVAN: Yeah, it’s partly us not being as creative as we can be when we think about AI. And that makes sense. Of course we want AI to go do our boring tasks. And we’re like, okay, how can it help me understand which emails in my inbox are important or not, or help me read this long document more easily? But many of the most interesting ways to use models are actually as a partner in this metacognitive process, for brainstorming, for ideation, for coming up with new ideas.
MOLLY WOOD: You’ve mentioned this idea that we could be more creative. From your vantage point, what are we missing?
JAIME TEEVAN: I think the changes on the way that we work, the way we get things done, the way we collaborate, they’re going to be really significant. I find maps is a really interesting metaphor here. We talked about how natural language is a really ancient technology. Maps are a really ancient technology as well. They’ve existed for millennia and have been extremely useful for path planning or, you know, delineating geopolitical boundaries. But pretty much the way we interact with maps hasn’t changed that much in the millennia that we’ve been using them, except for the fact that my kids can now no longer read maps. They don’t know what it is to look at them. And that’s because, actually, what’s happened with maps is we’ve taken them and digitized them. And do you remember when there was MapQuest, when we first started having to use MapQuest?
MOLLY WOOD: Oh, yes. And we would print it out, and we would have our MapQuest printout… [Laughter]
JAIME TEEVAN: And we would print it out, and we just used MapQuest like an actual physical map. It was just, it did the searching for us, and then we would go follow that. And so then what happened was, with GPS and our mobile devices, we started to be able to get turn-by-turn directions and see where we were going. And so, like, that’s a good kind of obvious way that technology makes maps better. And that’s the reason my kids can’t read maps. But that’s not a very creative use still. Like, printing it out, super noncreative; using it to get turn-by-turn directions, useful. But the interesting thing was once we’re all using maps, you know, what happens is all of a sudden we can see the paths that everybody is taking and we can start predicting traffic, and then reroute people based on where there’s a lot of traffic. That’s really interesting that you wouldn’t have necessarily seen coming. And then that gave rise to things too, like rideshare apps and entirely new ways to use geolocation. And so I think, similarly, we’re starting to see some of these new things, but I think we still have the rideshare apps to discover.
MOLLY WOOD: Amazing. Let’s translate that into this world of productivity and work more directly. Clearly, we know that individual productivity can go up, and I love the specific examples you have. How are you also, though, seeing group and division and even company-wide productivity increase if they do this right?
JAIME TEEVAN: We sort of take a bunch of biases to Copilot or any language model that we’re using—and we talked a little bit about that, where we try and do easy tasks. Another bias we take is we think about our individual tasks. When we think about Copilot, like, how do I do my email? How do I read these documents? But really, all of the work that we do is inherently collaborative. Writing a document is collaborative. It’s foundationally about how do you communicate ideas. The cool thing that Copilot does is it actually allows us to start turning our conversations into these durable artifacts. And we actually see customers who are, for example, having meetings to brainstorm new ideas and create artifacts. So it’s actually changing the way we collaborate with each other. And we were talking about grounding a little bit earlier, like, you can start thinking then about how do you help ground people to have good conversations? How do you actually help people work together better? And then we can use the knowledge that we create differently as well. One of the things that’s really interesting is we’re seeing the artifacts in the documents that people have become important, not just to share with other people so you can communicate with ideas, but actually become useful to ground a language model. We talk about the use of content like a document with a language model as grounding, just the same way we talk about grounding in a conversation with another human. And so we will go take these artifacts, ground a conversation. That’s an inherently different—like, the entire data estate of an organization essentially becomes grounding material that you can then start reasoning over with other people. Our work is essentially becoming having interesting conversations with our computer and with other people.
MOLLY WOOD: And so when you describe these meetings that have this, even if it’s an unspoken goal of creating these artifacts, are you saying that people are inviting Copilot into meetings? Like, they’re having meetings with people that they include Copilot in, and it all creates this sort of corpus of learning and understanding and collaboration?
JAIME TEEVAN: So when you are in a meeting, you have access to Copilot. You know, one of the things that I find is super useful is you join a meeting late and you want to know what’s happening, you can actually get caught up on that meeting by asking Copilot about what happened. We were talking earlier a little bit about how useful it is to have a safe space where you can sort of try out ideas. One of the things that I do in a meeting is to ask Copilot if something has been answered already. Even though I’ve been sitting in the whole meeting, I’ll be like, oh, what was said about this?
MOLLY WOOD: I zoned out a little. [Laughter]
JAIME TEEVAN: Yeah. And actually getting what was said summarized or rephrased a little bit differently really helps me be able to ask good questions and have better conversations with people. And actually, when you start thinking about the way that language models are changing our engagement with people, it’s really interesting. Teams meeting Copilot, like, that’s one of the most popular surfaces where Copilot shows up, both for use in meetings and also because it’s really nice after a meeting to be able to get a summary of what was discussed. And one of the things that’s sort of interesting is you would think the kind of default scenario everybody thinks of when they think of that, like, oh great, then I can go to fewer meetings because I don’t have to be there and I can just ask a few questions of what I missed. But it’s actually really useful, even if you were in the meeting to get a summary and be like, oh, that was what we talked about. Oh, I have some structure. I didn’t realize that those were the points that were hit.
MOLLY WOOD: Yeah. I mean, I could see that, just knowing people, I could see that being very useful in terms of a ground truth about a meeting. Like, this is what was said and this is the summary. It sort of feels like it could clean up corporate communication in a lot of really useful ways.
JAIME TEEVAN: Certainly having an artifact is, but in many ways, a meeting is sort of what we take out of it and how we interpret it as well. One of the things that’s interesting when you look at this research on summarization, is summarization on its own is almost never that useful. Because what you really need is a contextual, personalized summary. Like, you may take something different from a meeting than I take, and that’s not necessarily because we have a disagreement of the underlying facts that happened, but because there’s different things that matter, like, oh, I have to take from this meeting some action items and things I have to go do and you have to take from this meeting some new ideas that you’re going to think about. There’s sort of different ways that we use that underlying content, and that’s the reason, actually, just a raw summary isn’t the end goal. The cool piece is that ability to sort of have a conversation with the meeting. You’re essentially able to continue to interact with that meeting asynchronously. Teams always allows you to continue to interact in the chat before and after a meeting, but now you can actually interact with the conversation that happened. And in some ways, what we see is AI is starting to break down the boundary between synchronous and asynchronous communication, in that you can have a synchronous conversation and then continue that asynchronously later. And then what’s more, we were talking about documents and these digital artifacts, the digital artifacts you can actually start having synchronous conversations with. I can actually open up a document and ask questions of that document. That’s a much more synchronous type of engagement with a document than I was able to have previously.
MOLLY WOOD: Right. So a meeting is a living organism that goes on after—it’s the infinite meeting.
JAIME TEEVAN: Yep. Just what we all wanted, right? [Laughter]
MOLLY WOOD: In a good way. In a good way.
JAIME TEEVAN: This opportunity to have better, more interesting meetings where you get to focus on the important content, and having good ideas and using Copilot to help facilitate the conversation.
MOLLY WOOD: I mean, I personally am fascinated by the idea of agents. And I wonder how you see that changing as we then start to have AI agents who are interacting maybe on our behalf, how that levels up even the infinite meeting.
JAIME TEEVAN: AI agents are basically designed to handle tasks and processes autonomously, which means that they can work independently, generally, to achieve a specific goal. So they are designed to operate asynchronously. They are designed to communicate with other people and with other agents, and to learn from our interactions with them so that they can get better over time. Just as we were talking about, a lot of the early ways that people think about using AI was for individual tasks. And I particularly like how agents get us thinking about what AI looks like in more complex situations, you know, what does it look like to get something done that requires collaboration across people? How does it help facilitate and coordinate the work that you and I are doing together versus just the work that you’re doing alone?
MOLLY WOOD: Can you give me some examples of what that might look like? An agent helping you with that collaboration.
JAIME TEEVAN: We were just talking about meetings. So certainly you can imagine an agent joining a meeting that helps facilitate that conversation to make it as productive as possible, like, it helps tell us when we’re rat holing and move us on to another topic or help surface questions that weren’t getting resolved that we want to figure out. Another example is that agents are starting to manage long-term projects. So, for example, in Planner, AI can then go take a task, break it down and coordinate the work that needs to get done for that task across AI and across people.
MOLLY WOOD: Let’s zoom out a little bit. Tell us more about the research and how it’s driving this work.
JAIME TEEVAN: So research is really touching all aspects of product development right now, from figuring out how to build and fine-tune better models to figuring out actually the orchestration process of the model. And then of course we’re also thinking a lot about its impact on people and organizations and how they get things done.
MOLLY WOOD: Have there been any big surprises in the data?
JAIME TEEVAN: You know, the funny thing is things are changing so fast. Like, something that I would have told you was a surprise a month ago is now everybody’s like, duh, that’s obvious. [Laughter] Here’s an interesting thing that we’ve seen. So when you look at Excel, Excel is a really interesting place to think about using Copilot because it’s, most people’s early engagements with language models is in natural language, talking with it, making sense of ideas, and with Excel, you’re operating over numbers, and so we’ve done a lot of work to understand what does it mean to operate over numbers and understand and do data analysis with Copilot. But one of the things that we saw was that people were putting columns of text into Excel and asking Copilot to help them make sense of that text. So if you think about it, you can take survey responses from a bunch of different people in natural language, which used to be sort of like hard to understand, you’d have to read each one, and now you can start extracting themes and insights from that. It becomes really interesting when you start thinking about, what does that mean for collaboration? All of a sudden, you can take all of these different ideas from people, put it in an Excel document, and then reason over it. So we call this collective intelligence, the ability to gather insights from hundreds or thousands of people. Imagine if you want to understand what people in your organization are doing. And you ask them, what are your priorities for the coming quarter? And you capture that information. All of a sudden, you can see what your organizational priorities are, not just like as a top-down concept, but actually as a bottom-up concept that comes from the work that people are actually doing.
MOLLY WOOD: Just to understand this more specifically, if you can then put that into Excel, assign numerical values to it, you can really start to quantify what might otherwise be kind of fuzzy word-based data.
JAIME TEEVAN: So you can actually just say, in this column of priorities, what are the top priorities? And you can go directly to the high-level themes that are showing up from that data…
MOLLY WOOD: See, I’m totally like, how do you make it into a spreadsheet? And you’re like, no, no, no. Skip ahead, honey. Skip straight ahead to the outcome. [Laughter] I was not thinking creatively enough about how to employ AI here. That reminds me that you also mentioned earlier that AI is already changing the way people interact with each other. Can you say more about that?
JAIME TEEVAN: Yeah. There’s all this conversation around, like, 10 ways to prompt a language model. But I think looking forward, it’s going to be about how do we prompt people? You know, what are you thinking about? What was the big idea you had today? What is the big question you want to answer tomorrow or in a meeting? What is the thing you should talk about next to make this meeting particularly valuable? I remember early on when we were sort of first figuring out how to integrate language models into Office. We’re sitting around and we’re sort of in this small little V team trying to figure out the first kinds of features we’re going to bring. What’s the first iteration of Copilot going to look like? And there were two different teams that had fairly different perspectives on what they wanted to create. And they both wrote a document on how they envisioned Copilot to look. And it was hard, and there was like big discussions around what to do, and we just took the two documents and asked the language model to figure out what was similar and what was different, and what questions did we need to resolve. And it turned out, as much as it felt like there was a large gulf, there was so much similarity and we were able to then align on that similarity and be like, okay, these are the two points of contention that we need to figure out, and then we could solve that and move forward.
MOLLY WOOD: Wow. I mean, the implications of that are kind of staggering. Like, could we do that for America? That would be awesome.
JAIME TEEVAN: Yeah, that would be nice.
MOLLY WOOD: Amazing. Can I do a little lightning round with you, of questions about the future and yourself. I mean, I have to imagine you use AI in your work in some incredible ways. So give us some examples there.
JAIME TEEVAN: Well, so one is idea generation. You know, coming up with just different ways to express things. Language models are inherently generative. It is generative AI. They can generate great ideas. So if I’m like, oh, help me think of a better way to express this. Help me think of five clever ways to title something. I think that’s a lot of fun. I really like giving the model some prompts for how it should think. I’d be like, oh, come up with a title for this, have one of them be funny, have one of them be really insightful, have one of them be short and to the point. I think that’s really useful. The other thing that I really like using Copilot for is to get feedback. It’s amazing to get feedback, but if I send you a document and ask for your feedback, chances are you won’t get around to it for a couple days, and then you’ll send it back to me. With Copilot, I can get feedback right away. And actually that’s another place where providing a little bit of perspective, like, I’d like feedback on this from the perspective of someone in the field. I’d like feedback on this from the perspective of a science leader. I’d like feedback on this from the perspective of an engineer. It’s really good when writing hard emails to people to sort of get ahead of the ways that your mail might not land well.
MOLLY WOOD: Yes. In your experience, what use case for AI seems to be the biggest eureka moment for people?
JAIME TEEVAN: The one that I find the most calming is making sense of all of the noise that is in my head. I’m like, oh, I’ve got 27 thoughts running in 27 directions and I can’t figure it out. One of the things that I do is I start every morning asking Copilot, what’s top of mind for me right now? And it will comb through emails and meetings and conversations I’ve had and say, these are the five things that you’ve been thinking about recently. And that is just so calming, to just sort of have that, have it named and structured.
MOLLY WOOD: That’s such a powerful way to put that, because that’s exactly true. There is just something about an organizing principle that lets you take a breath and be micro productive. Right? Break it down to its component parts so I can move on.
JAIME TEEVAN: Right. Exactly. So you can see those pieces and actually, exactly as you said, then you can see the structure and you can be like, okay, I’m thinking about this thing and I can figure out what I need to do in this area instead of feeling like I’ve got 27 million things.
MOLLY WOOD: Amazing. Jaime Teevan is Chief Scientist and Technical Fellow at Microsoft. Thank you so, so much for the time today.
JAIME TEEVAN: My pleasure, Molly. Thank you.
MOLLY WOOD: Please subscribe if you have not already, and check back for the rest of season 7, where we will continue to explore how AI is transforming every aspect of how we work. If you’ve got a question or a comment, please drop us an email at worklab@microsoft.com, and check out Microsoft’s Work Trend Indexes and the WorkLab digital publication, where you’ll find all our episodes along with thoughtful stories that explore how business leaders are thriving in today’s new world of work. You can find all of it at microsoft.com/worklab. As for this podcast, please, if you don’t mind, rate us, review us, and follow us wherever you listen. It helps us out a ton. The WorkLab podcast is a place for experts to share their insights and opinions. As students of the future of work, Microsoft values inputs from a diverse set of voices. That said, the opinions and findings of our guests are their own and they may not necessarily reflect Microsoft’s own research or positions. WorkLab is produced by Microsoft with Godfrey Dadich Partners and Reasonable Volume. I’m your host, Molly Wood. Sharon Kallander and Matthew Duncan produced this podcast. Jessica Voelker is the WorkLab editor.
Microsoft is a technology company, a small local company, with few employees, no offices, and almost making no profit… >>
Please visit the firm link to site