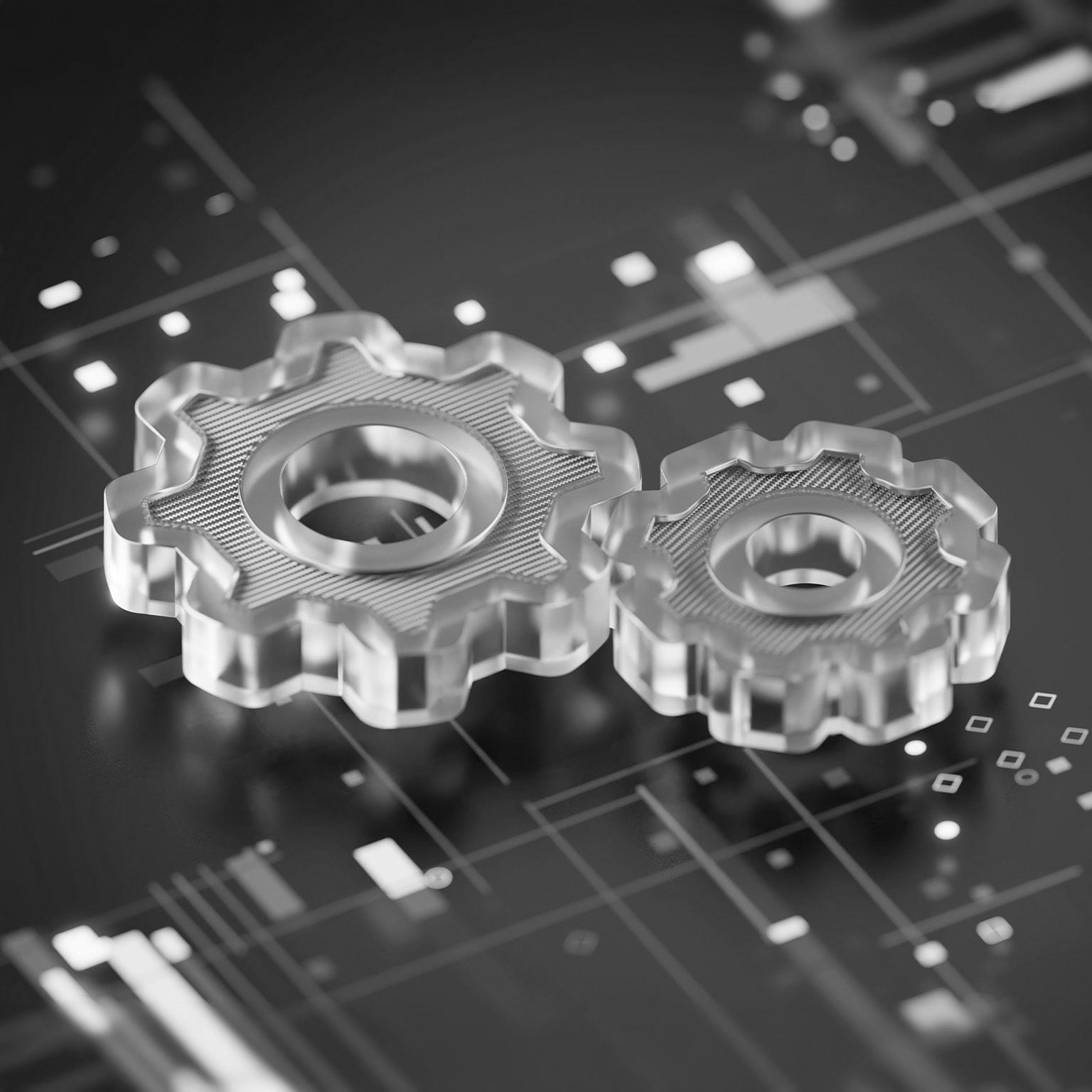
Modern machines are getting harder to maintain. Extra features, multiple sensors, advanced control systems, and sophisticated software all add cost and complexity to maintenance tasks. In industrial environments, that complexity is often compounded by the coexistence of equipment from different eras, different manufacturers, and different technology types.
As the work involved in maintenance becomes more demanding, maintenance functions are wrestling with other challenges. Workforce demographics are one, as older, more experienced maintenance personnel may take important knowledge with them when they leave a business. Skills mismatches are another. Advanced, software-heavy machines and modern techniques, such as condition-based maintenance, require maintenance teams to master new skills in digital and data analytics.
Time for a reboot
As the problems pile up, forward-thinking maintenance functions are searching for new ways to address cost, productivity, and skills challenges. Gen AI is emerging as a transformative solution for these challenges.
Gen AI tools use advanced machine learning models to accelerate data analysis, predict potential failures, automate routine tasks, and retain critical knowledge. Unlike previous generations of AI technology, gen AI systems can provide actionable insights and solutions that improve the efficiency and effectiveness of maintenance activities. Ultimately, that can help companies achieve higher reliability, reduce downtime, boost sustainability, and optimize the total life cycle costs of their machines and equipment.
Gen AI tools also have the potential to help maintenance functions address challenges around skills and the retention of knowledge within the organization. This includes:
- Increasing the performance and productivity of less experienced staff by automating routine tasks or using virtual agents to assist with maintenance planning, scheduling, troubleshooting, and repair
- Improving the accessibility of maintenance documentation with advanced search and summarization services, such as the automated generation of fault diagnosis steps
- Accelerating the integration and upskilling of new staff with automated onboarding, training, and collaboration systems
Some leading companies are already using gen AI to address long-standing challenges in their maintenance processes. The impact of these tools has been significant, and many have been deployed extremely rapidly, with lead times measured in weeks. Let’s look at two examples.
Automating failure modes and effects analysis
A company in the oil and gas sector is using gen AI technology to automate failure modes and effects analysis (FMEA) for thousands of items of equipment across its facilities. FMEA is a systematic method for identifying potential failures in a product or process, assessing their impact, and prioritizing corrective actions to mitigate risks. It can be challenging to implement because it requires detailed knowledge, cross-functional collaboration, and time-consuming analysis.
Like many organizations with complex production systems, the company had historically struggled to maintain accurate, consistent FMEAs for assets across multiple production sites. To address this challenge, the company built a custom gen AI system to ingest, code, and clean thousands of lines of data from existing FMEAs. The new system generated a comprehensive list of failure modes for each piece of equipment the company owned, linked to the most appropriate maintenance actions.
Armed with the gen AI tool, frontline maintenance teams are now evolving their maintenance strategies, adopting best practices from across the organization. The system continuously updates its library of recommended strategies based on the effectiveness of maintenance interventions elsewhere, helping the organization collaboratively improve overall maintenance performance.
Since implementing the gen AI FMEA tool, the company has seen a significant reduction in equipment downtime. Employee capacity has also increased because less time is spent manually creating FMEAs and related work orders.
A troubleshooting copilot for frontline production personnel
Another company, this time in the consumer goods sector, has built a gen AI copilot to help production operators resolve unplanned downtime in manufacturing machines. The copilot is designed to support staff in the execution of routine troubleshooting tasks without the need for input from specialist maintenance teams, significantly improving machine performance and overall line productivity.
The company trained its gen AI system using multiple data sources, including FMEAs, failure logs, and traditional troubleshooting tools such as fishbone diagrams and “five whys” analysis. For a given set of failure conditions, the system generates a step-by-step troubleshooting guide to help operators systematically exclude potential root causes. The diagnostic steps are presented in a clear, graphical format, using pictures and diagrams from machine operating manuals and factory standard operating procedures (SOPs). Following the guide helps operators find and fix problems quickly. Once a root cause is identified, the system offers a set of final recommendations designed to stop the recurrence of problems through preventive maintenance actions.
The gen AI copilot has transformed the effectiveness of operator-led maintenance interventions. Where operators once relied on trial and error to solve unscheduled breakdowns, the new approach helps them rapidly pinpoint the root cause, cutting unscheduled downtime by as much as 90 percent. Maintenance labor costs have fallen by a third, and technicians now have 40 percent more capacity since they rarely need to answer simple questions from operators or intervene to help resolve routine breakdowns.
Deploying gen AI at scale
While some maintenance organizations are already capturing significant benefits from gen AI, few have deployed these technologies across all the currently viable use cases. And many organizations have yet to begin their journey into gen AI–enabled maintenance.
Realizing the full potential of gen AI in maintenance is challenging for several reasons. These technologies are novel, requiring maintenance organizations to understand new technologies and avoid unfamiliar pitfalls. And gen AI is advancing extremely rapidly, requiring an agile approach to use-case selection, tool development, and continuous evolution.
Using the experience from previous waves of digital transformation and the deployment of gen AI in other sectors and functions, maintenance organizations can dramatically increase the odds of a successful gen AI transformation by putting four key enablers in place at the outset:
- A strong understanding of relevant gen AI use cases—together with their technology and data requirements—and common pitfalls that can hold deployments back
- A clear, organization-wide perspective on the capabilities and limitations of the maintenance organization, including key pain points and quantified improvement opportunities
- A dedicated team with the skills and capacity to develop, implement, and monitor the impact of gen AI use cases
- A robust change management infrastructure to support training, upskilling, and the promotion of a digital- and AI-receptive culture across maintenance and related operations functions
A maintenance transformation based on gen AI should focus on rapidly deploying promising use cases to generate value as early as possible while building the technical capabilities, infrastructure, and organizational culture needed to support the ongoing development, deployment, and continuous improvement of gen AI tools. Many organizations begin such a transformation with three distinct “sprints” conducted over approximately six weeks:
- Sprint 1: Conduct a gen AI value and readiness assessment to prioritize use cases and assess company readiness.
- Sprint 2: Design the target state, develop business cases for prioritized use cases, and evaluate gaps to be addressed.
- Sprint 3: Deploy one minimal viable product (MVP) gen AI use case with a detailed implementation road map to quickly unlock other prioritized use cases.
Strategic vision, capability building, and change management are the three key elements for ensuring transformation success. Long-term sustainability is supported by a fast scale-up process, focusing on involving people on the ground from day one.
Gen AI has the potential to revolutionize the maintenance sector by making operations more reliable, sustainable, efficient, and cost-effective. These technologies can provide tailored support for operators, optimize preventive strategies, address troubleshooting issues, and provide best practices for reskilling personnel.
The critical question for today’s maintenance organizations is not whether it is worth starting this journey but when. With a structured approach and the right tools, companies can begin to realize the value of gen AI in just a few weeks.
“Our firm is designed to operate as one—a single global partnership united by a strong set of values. We are equally committed to both sides of our mission: attracting and developing a talented and diverse group of colleagues and helping our clients create meaningful and lasting change.
From the C-suite to the front line, we partner with clients to help them innovate more sustainably, achieve lasting gains in performance, and build workforces that will thrive for this generation and the next.”
Please visit the firm link to site