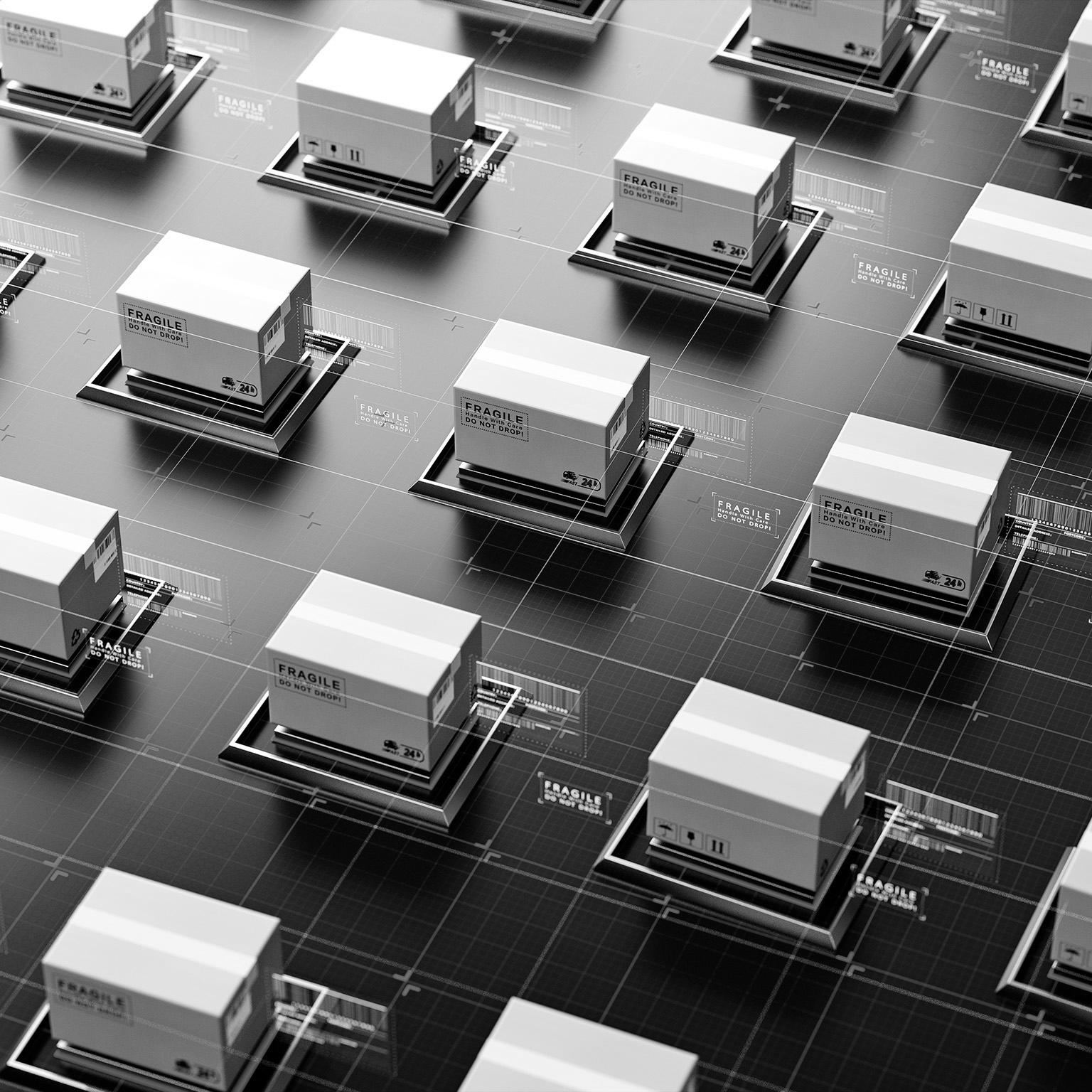
Companies are striving for end-to-end visibility, efficiency, and agility in their supply chains. Yet outdated infrastructure, fragmented data, and supply chain disruptions make these goals difficult to achieve. Can gen AI bridge the gap?
In this episode of McKinsey Talks Operations, host Daphne Luchtenberg speaks with Knut Alicke, author and leader of McKinsey’s Supply Chain Executive Academy; Alberto Oca, McKinsey partner and coleader of digital warehousing in North America; Asaf Somekh, cofounder of Iguazio, a McKinsey company; and Sanjit Biswas, CEO and cofounder of Samsara, to explore how AI-driven innovation is reshaping logistics, forecasting, and real-time decision-making.
The following conversation has been edited for length and clarity.
Sanjit Biswas: Once you have data about what’s happening in field operations, you can really start optimizing those operations. Some of this is fairly obvious. If you did a ride-along with every driver, you’d ask questions like, “Why are you taking that route?” or “Can we make the start-of-day and end-of-day processes faster?” It’s only when you can see it at scale that you realize how much operational-efficiency potential there is, and that happens in different companies in different ways.
Daphne Luchtenberg: Technology has been at the center of supply chain evolution, which has accelerated in recent years, with leaders investing heavily in digitizing and automating their supply chains and related logistics. Enter gen AI as one of the shiniest tools in the box. This much-talked-about technology has the potential to create a step change in supply chain efficiency. But with most digital investments failing to deliver value, supply chain leaders may be hesitant to make yet another significant gamble on tech.
With me today are my colleagues, Knut Alicke, author and leader of McKinsey’s Supply Chain Executive Academy in Stuttgart; Alberto Oca, coleader of digital warehousing in North America; and Asaf Somekh, cofounder of Iguazio, a leading AI and machine learning operations company acquired by McKinsey in 2023. The clip you heard at the start of the episode was from an earlier conversation with Sanjit Biswas, the CEO and cofounder of Samsara. We’ll hear more from this conversation later.
Since the launch of ChatGPT a little over two years ago, there’s been a lot of excitement about gen AI. But the technology has also been met with criticism that suggests its potential and value are overblown. Knut, you’ve spent a lot of time exploring AI’s potential with clients. What’s your take?
Knut Alicke: People tend to overestimate the impact of new, disruptive tech in the short term and underestimate it in the medium or long term. For me, it’s clear that we are at a moment similar to when the container was invented. The container completely changed how global supply chains operated, significantly increased efficiency, and enabled things that weren’t possible before. All our clients are exploring, testing, and trying to understand this new tech.
Daphne Luchtenberg: Asaf, you and your team built Iguazio on the premise of gen AI’s potential. What are you seeing in your work with companies today?
Asaf Somekh: AI has evolved a lot since the 1950s. When I was in university, I built my own models without the tools we have now that can automatically create models. As a company, we’ve been focused on AI for the past ten years. We’ve seen companies across all industries implement AI in their operations. It used to be machine learning and deep learning, implemented on manufacturing production lines through computer-vision-based quality assurance and in back-end banking operations.
Gen AI’s capabilities are leaps ahead of previous generations of AI. In the past, AI wasn’t good enough in certain areas to provide the expected value. Now, it’s opening up a lot of opportunities. The natural language makes this tech more intuitive, and higher-quality chatbots and copilots are changing the way many professions operate.
Daphne Luchtenberg: Asaf, what are you seeing in supply chains?
Asaf Somekh: The focus right now is on production pipelines. In advanced manufacturing, some companies use advanced technologies like silicon in their equipment. This is an area where visual inspection by humans is not as good as AI. Many people are working to implement AI in this area on a bigger scale than in past experiments with machine learning and deep learning.
But it’s important to say that not everything needs to be a gen-AI-based technology. We’re educating our clients on this, emphasizing that machine learning is good enough for some things. Gen AI is expensive in terms of compute resources. So we always recommend that people set up a flexible environment that combines traditional AI, like machine learning and deep learning, with gen AI. In distribution networks, a lot of people are trying to use AI to make routes more efficient and adjust people’s skills. The sky’s the limit when it comes to use cases in this space.
Daphne Luchtenberg: Building on that, what are some immediate use cases, Alberto?
Alberto Oca: Gen AI is revolutionizing the logistics industry. It’s poised to boost performance and trillions of dollars in operations, with roughly $190 billion in travel and logistics and $18 billion in supply chain operations.
Gen AI offers value-clearing opportunities across the entire logistics operations value chain. This ranges from core operations—activities like planning, optimization, warehousing, transportation, and asset maintenance—to support functions, like procurement, customer experience, back office, legal, and HR. In a nutshell, gen AI enhances various aspects of the value chain and leverages advanced AI models to create new, content-optimized processes and improve efficiency across logistics operations.
The benefits are impressive. For example, gen AI can significantly reduce the lead time for producing documentation by up to 60 percent. This includes auto-generating and consolidating shipping documents, identifying potential mistakes, digesting corrections, and reducing human errors and the workload of logistics coordinators by 10 to 20 percent.
Daphne Luchtenberg: It reduces the administrative burden, right? It enables people to get the right information quicker.
Alberto Oca: Exactly. Virtual dispatcher agents are also getting a lot of attention. These gen AI agents can augment dispatchers to assist drivers with troubleshooting and roadside assistance. For one company, a last-mile operator with a fleet of more than 10,000 vehicles, the implementation of virtual dispatcher agents led to $30 million to $35 million in savings, with an investment of just $2 million.
For warehouse insights, a virtual SME [subject matter expert] can synthesize findings from multiple systems in response to managers’ questions. This chatbot helps managers complete transactional activities in a way that optimizes performance.
And for customer experience, a three-way messaging platform can leverage gen AI and standard SMS text messaging to connect drivers, dispatchers, and customers in a single text conversation to improve efficiency and instantly resolve delivery-related issues. One carrier saved $3.5 million by implementing this platform for its fleet of over 150 vehicles.
Daphne Luchtenberg: So logistics is an exciting space for some of these opportunities. Now, let’s listen to a conversation we had recently with our guest from Samsara.
Sanjit Biswas: Let’s start with safety. DHL has been one of our customers and partners for many years. We work with their Express division and their Supply Chain division. They care tremendously about the safety of their front line. They have thousands of delivery drivers working every single day, around the clock, in all kinds of weather conditions. For them, making sure their front line is safe is paramount.
They already had safety coaching and safety programs, but they lacked operational data and context. In other words, what was happening out on the road? Were things like mobile phones and notifications distracting their drivers? They put our technology in the field four or five years ago and immediately saw a reduction in the number of events. Their drivers got into about 26 percent fewer accidents, year over year. Some of the accidents that were prevented were the most severe, so their costs decreased by 49 percent.
Daphne Luchtenberg: I love hearing about all these practical applications and their immediate return. Knut, we’ve been talking quite a bit about logistics and distribution. What about on the front end of the supply chain?
Knut Alicke: We worked on an interesting project for an order dispatcher. Multiple people were responsible for order management, with each of them delivering products to multiple customers. If there was a bottleneck, they ended up prioritizing one customer over the other. These rules are often grown rather than planned, and they usually aren’t well documented. So, we had the gen AI engine talk to each order manager to find out if their rule set was consistent. And if not, did it follow company strategy? Usually, it didn’t.
Then, we asked the gen AI engine to give feedback to the order manager and suggest allocations that follow company strategy, which was to maximize EBITDA. For example, we asked it to prioritize a fast-growing customer. It was interesting to see how well the engine applied this multilevel reasoning.
This made us think about gen AI as a team member, a new colleague that needs to be trained. We needed to give it the business context and provide feedback on its decisions so it could improve. This will be an interesting application going forward.
Daphne Luchtenberg: I love that. You’re teaching the bot to give advice so that the action is accurate the first time.
Knut Alicke: Exactly. And by interacting with planners, we get all of their knowledge and experience. The planner’s implicit knowledge can be made into a standardized, explicit process. This might sound worrying, but think about when a new person joins the team. It’s the exact same process they would go through. With this, you can define your standard procedures, identify whether you’re following them, and thereby enhance performance.
Daphne Luchtenberg: So you’re changing the process workflow. How much of that is intuitive to teams, and how much of that requires formal training and skill enhancement?
Knut Alicke: The teams find talking to the gen AI engine very intuitive. You need to overcome the first hurdle of people not knowing how to use it. But if the feedback, questions, and challenges are good, then people start to accept it. That’s what we saw—people got used to it and understood that their individual, local decision was not always the best decision.
Daphne Luchtenberg: There are always going to be people in the workforce who are curious and keen to learn, use, and fine-tune these tools. Is there a danger that other people will be left behind?
Knut Alicke: Definitely. The typical planner has been with the business for 30 years; they still know how to use a fax machine. We need to convince this group of people. We can only do that if we tell them that we need their knowledge to train and improve the gen AI engine. We need to make them curious to try out and play with the engine.
Daphne Luchtenberg: It feels like there’s a lot to consider, especially given the perceived cost to implement this technology successfully. But does it have to be complicated, Alberto?
Alberto Oca: It doesn’t have to be complicated or a huge investment. In many of the examples we’ve talked about, the investment was $1 million or less. The focus should be on creating a strong foundation that companies can build upon. The most important thing is to define that foundation and how you can expand on it.
Right now, companies that are investing early in gen AI are building capabilities to gain a competitive advantage. They focus on identifying high-potential use cases, like the ones we’re discussing; understanding priority opportunities and risks, like the ones Knut mentioned; and determining the necessary capabilities to capture the value. My recommendation for supply chain leaders is to think about the workforce of the future. The adoption of gen AI in logistics can change the workforce dynamic, shifting toward more value-adding tasks and a reduced manual workload.
We’ll have to convince the group of people Knut mentioned to shift gears. Identifying and prioritizing use cases to prove the value of gen AI will be critical. There’s going to be a lot of skepticism, so you’ll need to quickly prove the technical feasibility and estimate the business value. Then you can start developing MVPs, or minimum viable products, and scale up across the business. Remember that gen AI won’t solve all of a company’s problems. It’s just a digital enabler for organizations for business processes and operations.
Daphne Luchtenberg: Asaf, I want to bring you back in here because this touches on the tech architecture and underlying platforms. How should organizations and the CIO [chief information officer] think about upgrading and ensuring the tech platform is robust so that these new gen AI applications can really create value?
Asaf Somekh: Let me build on that foundation Alberto mentioned. We call it the AI factory. The idea is to build repeatable processes, not just one-off attempts or MVPs. Gen AI has made it easier than ever to launch pilots and proofs of concept. We’re seeing students without any data science knowledge create amazing applications within hours. But producing a robust gen AI application that performs 24/7, at scale, is a completely different story. That’s why we’re working with CIOs to create the right foundation.
Many tech executives mistakenly believe that if they’re not advanced cloud users, there’s no way they can use gen AI. But a lot of our clients are either self-regulated or working on-premises due to regulatory requirements. We’re helping them build AI factories that provide exactly the same capabilities as cloud providers like OpenAI, Microsoft, and Amazon. In fact, much of our work deals with helping companies implement hybrid architecture that keeps most of their high-impact applications on-premises, since that’s where their sensitive data sits.
When it comes to building the infrastructure, it’s not enough to create an accurate, working application. I’ve seen clients build gen AI applications they were satisfied with. But gen AI applications run on GPUs [graphics processing units], which are hard to find and expensive these days, whether you’re buying them for your on-premises infrastructure or paying for GPU hours in the cloud. Clients often realize that the cost of running these applications at scale is too high to justify the value they bring. This is where many projects get stuck. The right architecture needs to be in place, but there’s a big gap in the industry when it comes to how to use those GPUs. It’s one thing to get the application to work as intended, but quite another to do so cost-effectively. That’s why we’re focusing a lot on this area with clients.
At the end of the day, you want to create a centralized AI factory to ensure that you know which models you’re running in production and whether they expose you to any risks. There are new risks associated with gen AI, and while some people address them intuitively by keeping things on-premises, there are techniques to address those risks while scaling applications. At the same time, when developing applications, you want to provide a framework that can transition seamlessly into the production environment rather than stopping at the MVP stage. Often, projects will prove their value at the MVP stage but aren’t able to scale up because of cost or architecture.
Daphne Luchtenberg: It needs to be a forethought rather than an afterthought, right, Asaf?
Asaf Somekh: Absolutely. Some people say, “We’ll create the factory when we’re ready for scaling.” But you won’t be ready for scaling without it. Instead, you’ll have to go back several steps to fix your issues. So create it as early as possible, and it will pay off dramatically.
Daphne Luchtenberg: It sounds like supply chain leaders who are exploring what gen AI can do for their function are going to benefit from being very deliberate in their assessment of where and when to use tech. Most companies are still in the very early stages of their journey in gen AI. Can you paint a picture of what will be possible down the line?
Asaf Somekh: There is always hype for any new technology. What’s different about gen AI is that it made it all the way to consumers rather than staying B2B, which made the hype go through the roof. But like any technology, there is a hype curve. After the hype dies down, there’ll be some disappointment. But in the long run, it’ll create a lot of value.
In our experience working with clients, sometimes people get overexcited when thinking of use cases. Typically, the list starts with 100 ideas, but there needs to be some order and structure. That’s why we encourage people to focus on a domain and implement multiple use cases within that domain.
Copilots will be used a lot in the supply chain to improve the efficiency of the current workforce. You will be able to have a smaller workforce, and in the near future, we will see a lot of assistants that improve processes. Down the road, we will see autonomous applications.
Another hype term these days is agentic AI. In different industries, we’re building teams of agents that work with one another to complete repetitive jobs. That will come to supply chain, too.
Daphne Luchtenberg: Knut, what do you see as the future?
Knut Alicke: Typically, we find that our clients’ tech landscape consists of multiple planning systems and interfaces. Gen AI can encapsulate all this and be a natural-language interface that enables employees to make sense of planning decisions based on historical data. This will really boost the performance of supply chains.
Agentic AI is the next level. Right now, we have recommendations that enhance decision-making. With agentic AI, we could play that back into the systems. Agents that can place an order or transfer stock will further enhance supply chains.
And what do companies need to do? Be curious. Don’t wait to see how your competition uses it. There are no use cases out there that exactly fit your needs, so just start exploring. When you find a high-impact use case that works, scale it up fast.
Daphne Luchtenberg: Alberto, anything else you can add to the picture of the future for supply chain and logistics?
Alberto Oca: The future of gen AI and supply chain management is so promising. It has the potential to significantly enhance efficiency, decision-making, and operational performance. Gen AI is poised to augment the existing planning systems we’ve talked about, automate processes and repetitive tasks, and more importantly, provide valuable insights that will ultimately transform the supply chain landscape.
However, similarly to automation, it’s important to recognize its limitations and ensure that it complements human expertise and traditional software solutions.
Daphne Luchtenberg: That’s a nice thought to finish with, Alberto. It’s clear that gen AI isn’t going away and that there’s real value on the table to be captured. But what I’m hearing from all of you is that companies need to have a deliberate approach, be really clear about objectives, and have a unified approach across the senior-executive bench. Thank you all for this wonderful discussion, and special thanks to Sanjit for an equally engaging conversation [see “Safer, greener, faster: AI-powered supply chains in action,” forthcoming on McKinsey.com].
“Our firm is designed to operate as one—a single global partnership united by a strong set of values. We are equally committed to both sides of our mission: attracting and developing a talented and diverse group of colleagues and helping our clients create meaningful and lasting change.
From the C-suite to the front line, we partner with clients to help them innovate more sustainably, achieve lasting gains in performance, and build workforces that will thrive for this generation and the next.”
Please visit the firm link to site